ORNL sets its sights on a global challenge
Carbon is an exceptionally useful element, to say the least. That pound of sugar in your cupboard contains nearly seven ounces of carbon. Assuming you weigh 150 pounds, your own body contains 27 pounds of carbon. And the diamond on your wedding ring is made entirely of carbon.
Steel needs carbon, as do plastics and other polymers. Even your own DNA wouldn't exist without carbon.
But too much of this indispensable element in the wrong place can be a problem. That's what we face with the Earth's carbon cycle—a term that refers to the exchange of carbon among the planet's oceans, atmosphere, plants and soil.
Our atmosphere in particular has more carbon—in the form of carbon dioxide—than at any time in the last 3 million years, primarily due to the burning of coal, gasoline and other fossil fuels. In fact, the level of CO2 in our atmosphere has risen by a quarter in just the last 45 years.
Facing the problem head-on
This imbalance is the focus of an ORNL effort entitled “Balancing the Carbon Cycle”—a focus area that in the coming years will develop technologies to pull excess CO2 out of the atmosphere and other sources, as well as carbon contained in biomass and polymers, and turn it into valuable materials, thereby truly conserving carbon as a resource to enable production of valuable new materials.
“There is an incredible imbalance in the carbon cycle right now,” noted ORNL Corporate Fellow Bobby Sumpter, who is leading the effort. “It will require a lot of effort and a lot of creativity.”
The ORNL carbon focus is a recognition not only of the problems associated with carbon in the atmosphere, but also of the cost of squandering this valuable resource, noted Michelle Buchanan, the lab's deputy for science and technology.
“I was at a meeting back a few years ago,” she said, “and a guy from a major oil company got up and said if all we do is burn carbon, we're in trouble, because if we run out of easy-to-get, cheap carbon, you're not going to have polyester carpets, you're not going to have baby diapers, you're not going to have Saran Wrap, you're not going to have tables that have a plastic coating on the top. And so we need to think about saving those carbons and reusing them.”
ORNL is in a unique position to address the problem. With world-class experimental centers, the country's fastest supercomputer and expertise across the gamut of physical sciences and engineering, artificial intelligence and data analysis, the lab has the tools necessary to take a comprehensive look at the Earth's carbon cycle and devise strategies to bring it back into balance.
To be effective, Sumpter said, the project will require coordination among chemists, chemical engineers, physicists and biologists, as well as experts in artificial intelligence, machine learning and data analysis.
New strategies
The effort—a “science of the future” approach— will incorporate at least two new research strategies. First, individual projects will be tightly coordinated, from nanoscale explorations of new catalysts to scale-up of potential solutions.
“The difference between the science of the future approach and typical approaches,” Sumpter said, “is that we will take advantage of expertise that crosscuts everything from chemistry, physics, materials science and engineering, to computer science and applied mathematics, all the way through to ‘at-scale engineering’.”
With the carbon focus area, for example, efforts to create new catalytic materials and membranes will be undertaken with a close eye on how these materials would fit into a deployment of hundreds or even thousands of industrial facilities.
“We have the talent,” Sumpter said, “but we often tend to work on specific pieces of our own disciplines in isolation and don’t truly integrate the pieces together that are required to solve such a grand challenge problem. The old adage is highly pertinent: The whole needs to be greater than the sum of the parts.”
Second, the carbon focus area will be guided from day one by the lab's expertise in artificial intelligence, machine learning, data analysis and automation. Data from neutron scattering or electron microscopy experiments, for example, will be analyzed as the experiments are being conducted, and the computers may even tweak experiments as they are being conducted. And at least one lab will be robotically controlled.
“Right now, basically you take a measurement, and you go back to your office for a week and interpret it,” Buchanan said. "Then you go back and take another experiment, and you go back and interpret it.”
“This could be an iterative thing where you would get all this information together, and the computer would help you with that decision.”
Twofold approach
The carbon focus area will be divided into two coordinated thrusts.
Capture–separation–conversion. The first thrust area will develop technologies to capture carbon from the air, separate it from other gases and convert it into useful products or their precursors, all in one process (see “All-in-one carbon conversion,” page 12).
Led by ORNL physical chemist Shannon Mahurin, this will be largely a materials science and chemistry effort. Catalysts, membranes and other materials will have to be developed that are far more effective than anything currently in use and that can work collaboratively to enable simultaneous efficient capture, separation and conversion.
Under this thrust area, carbon dioxide will no longer be treated as a waste product to be disposed of in underground reservoirs. Instead, it will be used as a valuable feedstock for fuels, high-level polymers and other products.
Polymer chemistry. The second thrust area will focus on the chemistry necessary to turn CO2 and other carbon sources such as biomass and discarded (waste) polymers, into high-level polymers and other high-performance materials. (see “Making the most of captured carbon,” page 14).
Among the most promising potential products for the captured carbon are materials that perform well under extreme conditions or that can be used to manufacture aircraft and automobiles or as feedstocks for 3D-printing applications.
Milking data for all it's worth
Artificial intelligence and machine learning are this ORNL effort’s not-so-secret weapons. Each thrust area will be supercharged by the lab's computational expertise and by facilities such as the Summit supercomputer and the lab's Compute and Data Environment for Science, or CADES.
According to David Womble, ORNL's director of artificial intelligence programs, machine learning will boost this effort in critical ways.
One is the ability to sift through enormous data sets and identify potential cause-and-effect relationships that can lead, for example, to better materials or processes. While human researchers are reasonably good at verifying relationships that they know to look for, it takes the data-handling power of modern supercomputers and advanced data mining techniques to find that unlooked-for needle in a haystack.
“Inside these data sets there are complex correlations that humans are not able to extract—or even know to look for,” Womble said. “If we know to look for a correlation, then we often have a statistical process to find it. But if we don't even know what to look for, how do we start? That's where machine learning excels.”
Simplifying the problem
Another strength of artificial intelligence is the ability to create surrogates for especially data-intensive portions of computational models, ones where brute-force computations based on the laws of physics are not feasible.
“First-principles models are sometimes so complex that they are computationally intractable, even on modern supercomputers,” Womble said. “AI is able to take data—both experimental and from simulation—and create a faster-running model.”
The technique is especially valuable when a computation needs to address a wide range of scales. For example, Womble pointed to the creation of a unified system for capturing, separating and converting CO2.
For such a system, researchers will need models that range from the scale of molecules in a membrane or catalyst to the scale of air or liquid flowing through that membrane or catalyst. These scales will vary by at least six orders of magnitude in both space and time, from microns to meters and microseconds to seconds.
First-principles models that look at each molecule individually cannot handle that range of scales, Womble noted, and continuum models that average the important values are not accurate enough. But machine learning models developed using both experimental and simulated data—while they may not give detailed information at the microscale—can provide accurate and computationally feasible approximations at the macroscale.
Getting machines to communicate
The other technical challenge will be the development of seamless communication among the computers and experimental facilities, an effort known as Connected Smart-Instruments.
At least one chemistry lab involved with the project will take this approach to its logical conclusion. The Autonomous Robot-Controlled Chemistry Laboratory—or ARC Lab—will replace humans with robotic controls able to speed the process of conducting and analyzing experiments.
The ARC Lab enables robots to become researchers with direct access to integrated theory, simulation and modeling, allowing the lab to analyze data and adjust experiments accordingly.
Ben Mintz, who leads ORNL Federated Instruments, said the ARC Lab will focus on catalysis research.
“We're going to build a lab that incorporates a robot capable of moving between stations to perform experiments,” Mintz explained. “Anything that carbon cycle experimentalists need for catalysis—either liquid phase or solid phase—we're going to have the robot create them at a much faster rate.”
Mintz noted that the lab will have its own computer—called an Edge Analysis Center. Plans call for a small-scale demonstration of the lab by the end of 2021, which will be followed by a more complex lab setup in the following years.
Experiments will also benefit from ORNL’s high-performance computers, including Summit. With Summit talking to an advanced electron microscope, for instance, data can be analyzed as it's being collected.
“It should automatically inform the experimentalist,” said Neena Imam, who leads research collaborations for ORNL's Computing and Computational Sciences Directorate. “If we're doing things right, you should have the ability to perform analytics in real time.”
Imam noted that the Connected Smart-Instruments initiative is not limited to ORNL. Instead, DOE is moving to connect resources throughout the agency's national laboratories and other facilities.
Womble agreed that coordination between computers and experimental equipment can accelerate research.
“As you are running your experiment and doing your analytics, you may see that you need to change some parameters. AI-based controls may be able to change a parameter without having to wait for an entire experiment to run and then do postexperimental data analytics.”
The challenge, he said, is not to develop the needed expertise. That is already on hand. The challenge is simply to integrate the lab's world-class researchers and world-class equipment to work together concurrently toward a common goal.
The time for this coordination is now, experimentalists and computer experts strongly agree.
ORNL Separations and Polymer Chemistry Section Head Sheng Dai pointed to the powerful tools that will be employed by this effort, from supercomputing resources to world-class neutron scattering facilities to state-of-the art electron microscopes.
“The reason we think this is the right time and right place is that there's a lot of tools and expertise which previously were not available,” Dai said. "Previously, it seemed unthinkable that machine learning could allow you to provide some realistic guidance in terms of what kind of material space you need to look into.
“All these new tools allow us to dig more deeply into the fundamental processes happening in these systems, which may lead us to some really high-impact discoveries.”
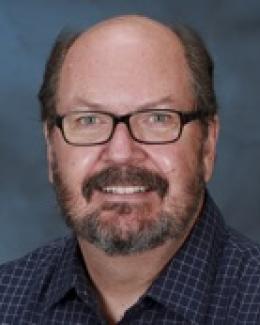