Participants at the AIRES workshop discussed topics ranging from experiment design to data analysis. Credit: Megan Jamerson/Oak Ridge National Laboratory, U.S. Dept. of Energy
Nearly 100 participants from government, industry and academia gathered at the Department of Energy’s Oak Ridge National Laboratory from January 22 to 24 for the inaugural Artificial Intelligence for Robust Engineering & Science, or AIRES, workshop.
Organized by ORNL, Sandia National Laboratories and the University of Tennessee, Knoxville, the AIRES workshop focused on applying artificial intelligence and machine learning to robust engineering. Specifically, speakers and attendees discussed designing, building, monitoring, controlling and predicting the behavior of physical systems, including large experimental facilities.
The workshop, which included a keynote address in addition to 17 full presentations and 16 “flash” presentations throughout five sessions, kicked off with opening remarks from Jeff Nichols, associate laboratory director for ORNL’s Computing and Computational Sciences Directorate, and David Womble, AI program director at ORNL and general chair of the workshop.
Traditional modeling and simulation techniques generate massive amounts of data that can be ideal for AI analysis if scientists can extract what they need. To provide context for the workshop, Womble framed this challenge as an opportunity for attendees to brainstorm creative AI solutions.
“The objectives for this workshop were to increase the strategic focus on AI for engineering and science and to identify barriers that demand additional investments, as well as to build new research partnerships,” Womble said.
David Brooks, director for General Motors Global Propulsion Systems R&D, described AI’s role in areas ranging from engine design to diagnostic tools in a keynote presentation titled “AI for Automotive Engineering.” AI, he said, could help make drastic changes to transportation and infrastructure that consider factors such as green initiatives and the increasing prevalence of megacities with populations exceeding 10 million people.
“AI has a huge part to play in the transformation of the automotive industry,” he said. “The sky is the limit here.”
According to Brooks, disruptors that will shape the industry include electric vehicles, connectivity between vehicles and other technologies, ride sharing services and autonomous vehicles. He discussed how AI could improve vehicle health monitoring systems that alert drivers to internal problems, as well as vehicle-to-vehicle communication capabilities that warn drivers of hazardous vehicles, dangerous road conditions, pedestrians and other external threats.
Brooks explained how—even with the most sophisticated equipment at hand—advances in AI might be necessary to help vehicle sensors interpret images from cameras and react appropriately to environmental factors.
GM collects invaluable data from vehicles in production and on the road to inform engineering development, safety features, and vehicle testing and validation. However, processing and analyzing all that data requires the most advanced diagnostic tools and computational resources. Brooks covered other data-related concerns such as training machine learning models and building datasets to evaluate vehicle performance during random, unpredictable scenarios.
“AI maps well-defined inputs to well-defined outputs, and there is no need for specialized dexterity, physical skills or mobility,” Brooks said. “But there is more data than ever for AI to learn from, and so much unstructured data can be hard to access and overwhelming to analyze.”
Subsequent sessions delved deeper into AI and machine learning capabilities that can predict system performance and determine potential causes of failure in the design, operation and maintenance of various systems. Topics discussed included early failure detection, lifecycle monitoring, data analysis in dynamic environments and integrating predictive tools into the development of robust systems.
The focus on data continued into an AIRES wrap-up conversation in which participants identified best practices—including more precise experiment design and better data capture strategies—to improve promising AI applications and overcome barriers to successful research.
“This workshop was both timely and unique,” said ORNL senior research scientist and AIRES attendee Olufemi Omitaomu. “On the one hand, we considered how AI techniques are already being used to make scientific discoveries in nontraditional areas. On the other hand, we discussed how AI techniques could transform systems including facilities, experiments and autonomous vehicles in the future.”
Funding for this workshop came from ORNL’s Laboratory Directed Research and Development program.
UT-Battelle LLC manages Oak Ridge National Laboratory for DOE’s Office of Science, the single largest supporter of basic research in the physical sciences in the United States. DOE’s Office of Science is working to address some of the most pressing challenges of our time. For more information, visit https://energy.gov/science.—Elizabeth Rosenthal
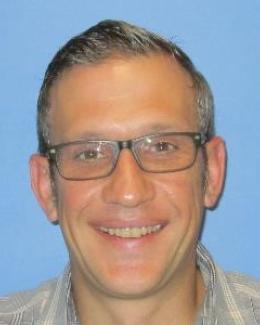