Research performed by a team, including scientists from the Department of Energy’s Oak Ridge National Laboratory, or ORNL and Argonne National Laboratory, has resulted in a Best Paper Award at the 19th IEEE International Conference on eScience.
The conference was held in Limassol, Cyprus in October.
Titled “Asynchronous Decentralized Bayesian Optimization for Large Scale Hyperparameter Optimization,” the award-winning paper uses the Polaris supercomputer housed at the Argonne Leadership Computing Facility, or ALCF, to present a new, overhead-reducing approach to Bayesian optimization, a technique for optimizing the hyperparameters of deep neural networks, or DNN.
ORNL’s Director of AI Programs and Distinguished R&D Scientist Prasanna Balaprakash shared the award with lead author Romain Egele, a research aide at Argonne; Isabelle Guyon, director of research at Google, and Venkatram Vishwanath, data science team lead at the ALCF.
Bayesian optimization is used as a common solution to black box optimization problems that arise in various machine learning tasks, but its most common implementation, the “single manager, multiple worker” utilization approach, suffers from inefficient scaling. Scaling problems can hinder accuracy and convergence speed, as demonstrated by the gains the new, decentralized method introduced in the paper offers relative to central management — making solutions increasingly important across scientific disciplines as exascale systems emerge as the new standard for high-performance computing.
“Automating the development of scientific machine learning models is one of the key challenges in trustworthy AI, and our research takes a significant step towards addressing this challenge,” said Balaprakash. “It enhances hyperparameter optimization efficiency in deep neural networks, crucial for developing more accurate and reliable AI models. This approach leverages high-performance computing, significantly reducing computational overhead and accelerating the model development process, opening new possibilities for AI applications in scientific research that align with the advancements in exascale computing”.
Use cases include simulator calibration, scientific simulation optimization, automated search of machine learning pipelines, software tuning and tuning of neural network architectures and hyperparameters.
Comparing their approach with traditional centralized Bayesian optimization, the researchers examined the benefits of employing decentralized architecture through an empirical analysis.
“Our new Bayesian optimization method improves solution quality and the speed of hyperparameter optimization via optimized utilization of resources in the evaluation of black box functions. This is achieved through decentralized management, as opposed to using a single central manager,” Egele added. “As researchers begin to transition to exascale systems, decentralized-management setups stand to benefit a wide range of use cases including fine tuning foundation models for science.”
The award is just the latest accolade for ORNL’s AI Initiative, which Balaprakash oversees.
The initiative’s cross-disciplinary foundational framework seeks to ensure that AI systems are secure, trustworthy and energy efficient. The security dimension covers aspects such as alignment with scientific goals, cybersecurity measures and robustness against failures.
Trustworthiness is ensured through rigorous validation and verification processes, uncertainty quantification and causal reasoning methodologies. Energy efficiency is achieved through scalable solutions, edge computing and a co-design approach that optimizes both software and hardware resources.
The laboratory boasts more than 300 staff actively using AI to tackle some of science’s most difficult problems, research that has garnered more than ten patents. Its flagship computer, Frontier, is not only the world’s fastest but was architected specifically in part to facilitate energy-efficient and scalable AI-based algorithms and simulations.
UT-Battelle manages ORNL for DOE’s Office of Science, the single largest supporter of basic research in the physical sciences in the United States. The Office of Science is working to address some of the most pressing challenges of our time. For more information, please visit https://energy.gov/science.
Please click here to read the original story by Argonne National Laboratory.
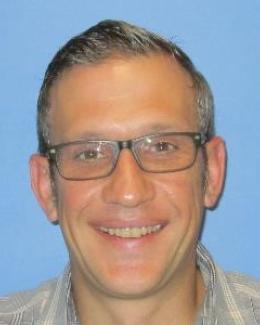