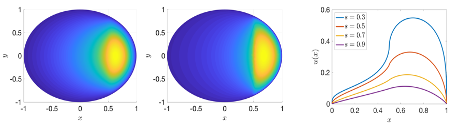
The Science
A learning-based approximation strategy has been developed to accelerate parameter studies for non-classical models of diffusion. The method relies on rigorous understanding of model behavior as a function of the parameter choice. It enables significant computational speed-up by inferring the most important information in advance (offline), and then constructs a cheap surrogate model for fast evaluations (online). In addition, the approach guarantees accuracy with certified error bounds.
The Impact
Non-classical models of diffusion are being used to advance our understanding of materials and transport processes and to improve image analysis. While these models reflect certain phenomena better than their classical counterparts, they also come with a higher computational cost. By reducing computational time, the proposed strategy enables practical application of such models for large-scale tasks such as optimization, design, and uncertainty quantification.
PI(s)/Facility Lead(s): Olena Burkovska
Publications: [1] O. Burkovska, M. Gunzburger, “Affine approximation of parametrized kernels and model order reduction for nonlocal and fractional Laplace models” accepted to SIAM J. Numerical Analysis (arXiv:1901.06748v2).
Funding: DOE ASCR AEOLUS (75%), DOE ASCR Householder Fellowship (25%).