We suggest and implement an approach for the bottom-up description of systems undergoing large-scale structural changes and chemical transformations from dynamic atomically resolved imaging data, where only partial or uncertain data on atomic positions are available. This approach is predicated on the synergy of two concepts, the parsimony of physical descriptors and general rotational invariance of non-crystalline solids. The first concept is implemented here via the classical variational autoencoder applied to semantically segmented atom-resolved data, seeking the most effective reduced representation for the system that still contains the maximum amount of original information. The second concept is implemented using the rotationally invariant extension of the variational autoencoder. This approach allowed us to explore the dynamic evolution of electron beam induced processes in a silicon-doped graphene system, but it can be also applied for a much broader range of atomic scale and mesoscopic phenomena to introduce the bottom-up order parameters and explore their dynamics with time and in response to external stimuli. The interactive Jupyter notebook that goes through the analysis described in the paper is available for fully traceable analysis and applications to the new data.
This approach is further extended towards analysis of phase transitions. Traditionally, phase transitions are explored using a combination of macroscopic functional characterization and scattering techniques, providing insight into average properties and symmetries of the lattice but local atomic level mechanisms during phase transitions generally remain unknown. Here we explore the mechanisms of a phase transition between the trigonal prismatic and distorted octahedral phases of layered chalogenides in the MoS2 ReS2 system from the observations of local degrees of freedom, namely atomic positions by Scanning Transmission Electron Microscopy (STEM). We employ local crystallographic analysis based on machine learning of atomic environments to build a picture of the transition from the atomic level up and determine local and global variables controlling the local symmetry breaking. In particular, we argue that the dependence of the average symmetry breaking distortion amplitude on global and local concentration can be used to separate local chemical and global electronic effects on transition. This approach allows exploring atomic mechanisms beyond the traditional macroscopic descriptions, utilizing the imaging of compositional fluctuations in solids to explore phase transitions over a range of realized and observed local stoichiometries and atomic configurations. Finally, we extend this approach towards the identification of the generative physical model, i.e., lattice Hamiltonian that can be used to generate atomic configurations and make testable predictions at a range of concentrations and formation temperatures.
DOI: 10.1126/sciadv.abd5084
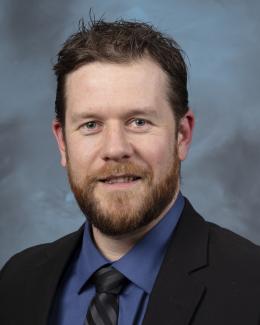