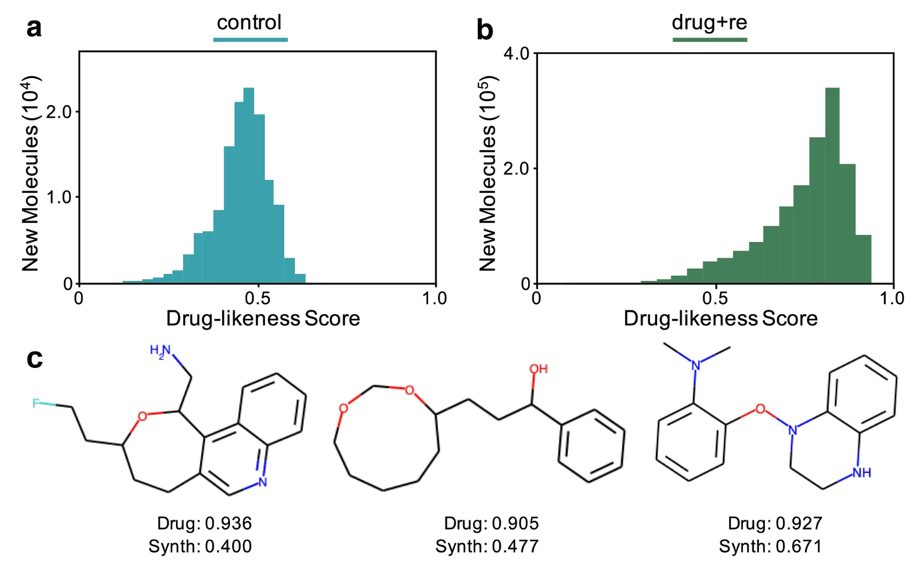
The Science
Generative machine learning models, including GANs (Generative Adversarial Networks), are a powerful tool toward searching chemical space for desired functionalities. Here, we have presented a strategy for promoting search beyond the original training set using incremental updates to the data. Our approach builds on the concepts of selection and recombination common in Genetic Algorithms and can be seen as a step towards automating the typically manual rules for mutation.
The Impact
Our results suggest that updates to the data enable a larger number of compounds to be explored, leading to an increase in high performing candidates compared to a fixed training set. Introducing replacement and recombination into the training process empowers the use of GANs for broader searches in drug discovery.
PI/Facility Lead: Debsindhu Bhowmik
Funding: DOE ASCR, ECP
Publication for this work: A. E. Blanchard, C. Stanley and D. Bhowmik; Using GANs with adaptive training data to search for new molecules, J Cheminform (2021) 13:14; DOI: https://doi.org/10.1186/s13321-021-00494-3