National Security at ORNL
ORNL researchers are a step closer to creating a facial image from a DNA sample, using a novel method that assesses facial scans and compares them to genetic markers.
The technology could improve identification at national borders and help local law enforcement identify crime suspects and unknown remains. ORNL’s DNA2Face project has as its long-term goal the ability to predict facial structure using genetic data such as a small blood or tissue sample.
“These days if you get a genetic sample from a crime scene, basically all you can do is apply it to some databank of samples, and we don’t have a lot of those. But if you can take that genetic material and make a face prediction, then you can run it against a database of faces, which we have a lot more of,” said Ryan Tokola, principal investigator in ORNL’s Imaging, Signals, and Machine Learning Group.
Researchers first defined a reference, average face mapped with tens of thousands of 3-D points. The reference face was then aligned with a similarly mapped facial scan. Comparing the two created correspondence vectors—essentially a measure of the differences between the reference face and the scanned face.
Once the correspondence vectors were established, the researchers applied a statistical technique called principal component analysis that transformed each correspondence vector into a relatively small set of numbers, which provided a very compact representation of the face. They then performed a genome-wide association study—a statistical approach to finding genomic variations that are associated with a particular trait—using DNA samples obtained from the subjects of facial scans. The results of the study showed several strong connections between DNA and facial features by comparing principal component analysis scores to DNA markers.
The ORNL researchers found 30 significant mutation locations and more than 5,000 locations that are potentially significant. In comparison, an earlier study without ORNL’s statistical method found only five significant locations.
Demographic characteristics such as ethnicity, gender, and age were taken into account for the subjects included in the research. The most significant cluster of variations was found on chromosome 3 on the RAF1 gene, which is known to affect craniofacial shape. Analyzing select portions of the face could result in the discovery of more genes that directly influence facial shape, Tokola noted.
“If you’re trying to identify someone, it’s a lot easier to do with an image of their face, which you can then feed into a face recognition database or crowdsource,” Tokola added. “You might put that image on the news and ask, ‘Have you seen this person?’ You really can’t say, ‘Have you seen these genes?’”
See also:
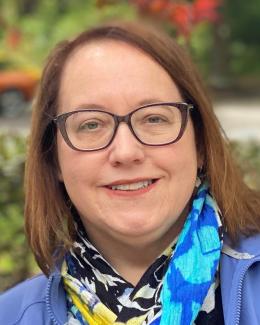