Guannan Zhang. Image credit: Carlos Jones, ORNL
"When I was an undergraduate, my major was mathematics,” Guannan Zhang said, “but when I went to graduate school, I got into programming and other aspects of computing, so it was very natural for me to pursue a Ph.D. in computational mathematics.”
His ECRP proposal, “Advanced Uncertainty Quantification Methods for Scientific Inverse Problems,” continues in a similar vein, focusing on developing better ways to determine the reliability of scientific inverse problems — the process of looking at the effects of an occurrence and calculating its causes through a process called inverse sampling.
“A scientific inverse problem uses measurements that are obtained from experiments to infer unobservable quantities that we are interested in,” Zhang said. “For example, when scientists at the laboratory’s Spallation Neutron Source conduct a neutron scattering experiment on a sample of material, they often try to determine its unobservable structure by analyzing the observable neutron scattering patterns that are produced when a beam of neutrons passes through the sample. That’s a scientific inverse problem.”
Inverse sampling can have an advantage over measurements taken in experiments that are subject to data noise and uncertainty; however, the sampling technique also presents the challenge of data uncertainty. Zhang’s research will address this and other inverse sampling–related challenges by building deep neural networks, a kind of artificial intelligence. The networks will learn to conduct inverse sampling more efficiently and accurately while solving inverse sampling problems in a range of disciplines.
“There are a lot of methods that can give you an answer to inverse problems,” Zhang said, “but because you don’t have enough prior knowledge to verify whether or not this answer is right, the biggest question is, should you trust this answer or not? The main idea of my proposal is to develop methods that will enable us to quantify the confidence you can have in the answer to an inverse problem. When the scientists use my method to solve an inverse problem, they will not only get an answer, but they will also get the confidence level of that answer. This will help them make more reasonable and informed decisions.”
Zhang noted that his five-year project will concentrate on fundamental research aimed at improving uncertainty quantification. Scalable implementation will be follow-on work.
“In five years, we will be able to develop deep neural networks. After that, we hope to accelerate their performance on bigger computers,” Zhang said. “We need to use AI to determine the best way to go from the observable to the unobservable. If we didn’t use AI, we would have to try all the possible solutions, which is not computationally affordable. Even with the biggest supercomputer, you couldn’t really do this by brute force. You have to search for the most likely solutions in a smart way. That’s where AI can help.”
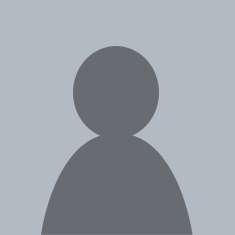