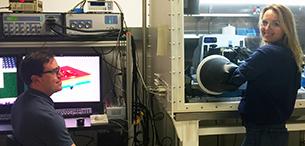
The Titan scanning transmission electron microscope at ORNL can produce tens of gigabytes (GB) of data within an hour and could potentially operate at much higher data generation rates. (For comparison, 10 GB alone is about 10 times more data than many smart phone users consume each month.)
“The tremendous progress in high-resolution imaging techniques now allows scientists to make direct measurements of the positions and distances between atoms, providing information not only about how they are arranged but also about how they interact,” said Sergei Kalinin, director of ORNL’s Institute for Functional Imaging of Materials (IFIM). “The bond lengths and angles we derive can give us a lot of information about the material’s useful functionalities and how they might be manipulated on the atomic levels to better perform these functions.
“But in many applications, it typically takes weeks for researchers to process this data and months— sometimes years—to understand it.”
The disparity between image collection and analysis can lead to a lot of stones left unturned, and it slows the pace of research into new or improved materials that could be used for applications like high-temperature superconductors, energy-efficient photovoltaics, and rare earth substitutes, among others.
That’s why ORNL material sciences researchers are collaborating with computer scientists in ORNL’s Compute and Data Environment for Science (CADES) within the lab’s Computing and Computational Sciences Directorate. Access to the powerful computing, advanced algorithms, and computational tools available through CADES can significantly reduce the amount of time it takes researchers to study data and report new discoveries, and through the collaboration between domain science experts and CADES computer and data science experts, researchers are creating a processing and analysis workflow for the expansive scanning probe and electron microscopy data generated at CNMS.
By establishing a rapid, automated method for image analysis, researchers are hoping to find new patterns: What structural properties do superconducting materials share on the atomic level? If a defect occurs during manufacture in one type of material, will it occur in another? How will a certain chemical reaction affect atomic bonds differently in various materials? These and similar questions require making connections, and until now, they have often relied on communication within niche communities of domain science experts.
What Kalinin and ORNL team members Rick Archibald and Albina Borisevich would like to see is “big data” become “deep data,” then “smart data,” through computerized analytic techniques such as pattern recognition and machine learning that make meaningful connections as rapidly as the data is being collected. Beyond putting more data to good use, smart data techniques can free up scientists’ time to answer fundamental questions in their research domains and innovate new materials and applications.
IFIM and CADES displayed the first data flow for rapid, systematic analysis of a large-scale imaging dataset at the SC14 supercomputing conference in New Orleans in November 2014.
The data demonstration pipelined data from the Titan scanning transmission electron microscope to ORNL’s Titan supercomputer, a Cray XK7 capable of 27 petaflops, or 27 quadrillion calculations per second. (The Titan names are coincidental.) Twelve hundred nanometer-scale images of perovskites—a family of oxide minerals commonly used in energy applications such as fuel cells, photovoltaics, and semiconductors—were atomically resolved and analyzed.
Both structural data points, related to atomic composition and arrangement, and spectral data points, related to energy measurements, are calculated during imaging to provide information about the atomic arrangement and dynamics of the perovskite samples. During the demo, images representing more than a million atoms across 300 million pixels were analyzed as part of the computational workflow to extract structural information, then were correlated with spectroscopic data sets via multiresolution data matching, ultimately aiming to identify structure–property relationships about defects found in the samples.
Scalable imaging processing on the Titan supercomputer transformed this big data set into what researchers call “deep data,” or data analyzed using physics-based interpretation to identify properties with which scientists are familiar. This data was compared across the 1,200 samples inputted to reduce the data sets to a smaller number of averages representative of each type of perovskite.
“We developed a custom code that identifies atoms on multiple slices and registers these atoms to the material structure as the structure evolves over time,” Archibald said, “Using this workflow on Titan allows us to reduce the compute time from many hours, or even days, to a few minutes.”
One of the team’s long-term goals is to harness computational methods to transform this deep data into smart data, which makes a connection between the data being processed and analyzed and established data in the form of theoretical models or database collections to build on existing knowledge. To make meaningful connections between existing information about perovskites during the SC14 demonstration, the team incorporated the reduced data from the Titan supercomputer into an electronic structure model running on the Edison supercomputer at the National Energy Research Scientific Computing Center. This theoretical model used the observational data to predict the functional properties of the perovskites imaged.
Because perovskites have been researched widely and scientists already have a good understanding of their structure, dynamics, and properties, the team was confident the workflow was successful and provided accurate information from the images.
“In this case, the perovskites are well-studied,” Kalinin said. “However, once our algorithms are verified against perovskites, we can use these tested algorithms against lesser understood materials to learn new and, as of yet, unattainable information.”
While the IFIM smart data project is in its early stages, Kalinin ultimately envisions genomic libraries of structure–property relationships on the atomic level that are expanded and explored through machine learning like that carried out in the CADES environment. Ready access to such libraries would allow researchers from different domains and research applications to explore material properties at new depths and ranges that could lead to transformative methods in materials research and design.
—Katie Elyce Jones