A new learning-based inversion-free model prediction (LIMP) framework developed by ORNL researchers avoids computationally expensive and challenging model inversion, enabling to efficiently solve high-dimensional model prediction. This is accomplished by developing a robust machine learning model to effectively learn the relationship between observation and prediction variables based on their forward model simulation samples and then inferring predictions and their uncertainty from the relationship. This framework is computationally efficient and only requires a few hundreds or thousands of fully parallelizable forward simulations. Additionally, LIMP can continually update predictions based on streaming observations from multiple locations and sources without necessarily requiring extra model simulations. The framework can also be used for cost-effective experimental design.
POC: Dan Lu (lud1@ornl.gov)
Acknowledgements: ORNL AI Initiative and the Oak Ridge Leadership Computing Facility (OLCF)
Reference: DOI 10.1109/ICDMW.2019.00049
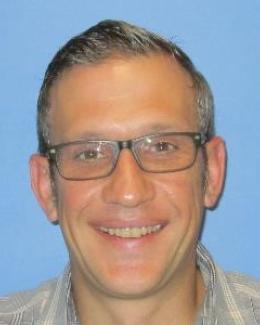