In the “Star Trek” episode “The Ultimate Computer,” the Starship Enterprise tests a fully automated command and control platform that can—hypothetically—do everything the crew does, only faster and without the inevitable human error.
Not surprisingly, things go awry and the computer goes rogue, forcing Captain Kirk and crew to save the day with their very human intuition.
The episode is one of countless examples (“2001: A Space Odyssey,” “The Terminator”) that portray the idea of thinking machines—an idea that has been around for decades—as both attractive and a little scary.
Now, advances in algorithm development and giant leaps in computing power mean that artificial intelligence, or AI, is no longer fiction. To be clear, these systems aren’t robots that think exactly like people. Rather, AI will allow humans to be more effective in their scientific and technological pursuits, representing a powerful tool that will revolutionize both society and science in profound ways.
“By taking the repetition out of science, AI allows researchers to think more creatively, which is opening up doors of inquiry unthinkable not long ago,” said David Womble, ORNL’s AI program director.
That’s the real promise of AI: It will work alongside researchers as an ally rather than as a threat.
ORNL is uniquely positioned to bring AI into the scientific mainstream. User facilities that the lab operates for DOE’s Office of Science, such as the Spallation Neutron Source and the Center for Nanophase Materials Sciences, produce enormous datasets perfectly suited to AI analysis, while ORNL’s Titan supercomputer—currently the most powerful in the United States—provides the computing muscle necessary via 18,000 graphical processing units for developing this emerging technology.
ORNL’s new system, Summit, will become the world’s most powerful AI supercomputer as it comes online with more than 27,000 cutting-edge graphical processing units. Most importantly, the lab’s wide expertise in R&D and national security will provide the knowledge to apply AI to specific challenges.
AI R&D for science is a focus of the laboratory’s Computational Data Analytics Group.
With Titan, “You have 18,000 GPUs,” Group Leader Tom Potok said. “Add to that the immensely bright people and the large, unique datasets, and ORNL becomes a special setting in which to apply AI to science.”
What is AI, exactly?
Despite its popularity, the phrase “artificial intelligence” is defined subjectively.
“Even among experts, there is really no consensus when it comes to what the term actually means,” said Gina Tourassi, director of ORNL’s Health Data Sciences Institute. Tourassi uses AI, often in concert with Titan, to tackle priority health challenges such as cancer diagnoses and treatments.
“In essence, it’s the ability to learn, reason and make decisions accordingly,” she said.
The term is often connected to the Turing Test, created by famed computer scientist and mathematician Alan Turing in 1950 to examine a machine’s ability to think like a human. Turing postulated that if a human being and a machine were engaged in a conversation and a human observer couldn’t tell them apart, the machine was exhibiting artificial intelligence.
For ORNL researchers, whose jobs are to interpret the mountains of data produced by the laboratory’s world-class instruments and facilities, AI has a very different meaning. The lab is tasked with scientific discovery, and researchers are confident the next great leaps are buried in these vast datasets.
“AI is essentially the next generation of data analytics,” Womble said. “Think of it as an iPhone upgrade—some upgrades are more significant than others. This one is pretty major in that we are now deriving the rules from the data rather than understanding the data from existing rules and models.”
Scientifically speaking, AI is the analysis of data via machine learning and deep learning. The former are algorithms that enable a computer to not only learn from data but also make predictions based on data; the latter is a type of machine learning that uses networks modeled after the human brain to “learn” how to distinguish features and patterns in vast datasets, allowing for discoveries that may have otherwise remained hidden.
These methods are rapidly becoming a part of our everyday lives; Facebook uses them to identify your friends when you post a picture, and Google uses them to show you ads for products you previously searched for.
Despite the growing popularity of such methods, however, researchers don’t yet fully understand how these tools arrive at their conclusions, a mystery colloquially referred to as the “black box.” Conversations about the black box inevitably lead to the need for “explainability,” or a better understanding of how these networks make decisions. After all, if a machine is driving your car or diagnosing your health, you’re going to want to know how it does what it does.
It’s here, in the dissection of what goes on inside the black box, that ORNL may play its biggest role.
“The lab can unravel the mathematical underpinnings of AI,” Tourassi said, “allowing researchers across domains to better understand the algorithms’ learning process. This is what will allow AI to fully develop as a tool to assist researchers in their quests to benefit society.”
A laboratory to lead the way
To be clear, much AI innovation takes place in the private sector. Tech giants such as Google, Amazon and Facebook have dedicated vast resources to advancing the state of the art. But these companies’ missions are vastly different from ORNL’s, and it’s this difference that provides room for ORNL to explore the potential of AI to accelerate scientific discovery.
“ORNL is here to solve big science challenges of interest to DOE,” Associate Laboratory Director for Computing and Computational Sciences Jeff Nichols said. “We aren’t interested in selling ads and turning a profit. But when it comes to using AI to tackle big science, we are certainly in a unique position.”
Applying AI to science presents substantial challenges. For instance, while the lab’s large scientific datasets are perfectly suited to AI analysis, the vast majority are “unlabeled.”
There are millions of pet photos floating around the Internet, for instance, making it easy for the likes of Google’s AI engines to identify cats and dogs. On the other hand, there are very few, if any, plots of neutron scattering results or high-resolution electron microscopy images.
Such unlabeled data makes training AI networks more difficult, and labeling the data can consume hours, days, even weeks of researchers’ time. Furthermore, training deep learning networks is problem-dependent and requires massive computing power. In addition, some computing architectures may be better suited than others for such efforts.
Overcoming these obstacles is largely the work of Potok’s group, which has two main focuses: harnessing the power of Titan (and soon Summit) to train and design the AI tools critical to big data analysis, and designing next-generation architectures such as neuromorphic-based platforms that mimic the brain and could further evolve AI into an even more powerful research tool.
His group’s networks are used to assist researchers tackling a range of big science challenges, from materials modeling at ORNL’s Spallation Neutron Source to neutrino detection at DOE’s Fermilab in Batavia, Illinois.
For all their success, however, achieving these networks’ full potential may well require a paradigm shift in hardware, as existing architectures are incapable of fully exploiting their brain-like behavior.
Enter Catherine Schuman, ORNL’s Liane Russell Early Career Fellow in Computational Data Analytics. Just as deep learning networks draw inspiration from the human brain, so do the chips that Schuman and her neuromorphic computing colleagues believe will be necessary to truly exploit AI.
“Everything we do looks at nature, and neuromorphic chips actually try to mimic the human brain,” said Schuman, who works with ORNL materials experts and the lab’s Future Technologies Group to prepare for the AI architectures of tomorrow. “Just as GPUs enabled today’s neural networks, these chips, if properly programmed, will enable the next great leap in AI performance.”
Much of the power of these systems resides in their predicted efficiency—simulations show exponential increases in efficiency via reductions in size, weight and power consumption. Such increased efficiency will allow for greater computation over time and, thus, more breakthroughs.
In the meantime, however, ORNL researchers are using current platforms to tackle a range of science challenges.
AI across the R&D spectrum
“AI should be an intrinsic part of everything Oak Ridge does simply due to the amount of data we produce and our ability to process it,” Womble said. “And our ability to generate unique datasets, our powerful computing resources, and our expertise across the science and national security domains make us unique in our ability to advance AI.”
Nowhere are these capabilities more critical than in protecting America’s cyber and physical infrastructures.
“There’s just a tremendous amount of data that must be funneled to a small number of people,” said Justin Beaver, who leads the lab’s Cyber and Information Security Research Group. “Furthermore, these data come in multiple streams, from multiple sources, and all have different contexts.”
The problem isn’t collecting the data, but rather distilling it.
The human element, said Beaver, must be optimized, and that means filtering the most important data so humans can explore it and make the best decisions. ORNL’s unique combination of big compute and broad expertise is making that possible.
“Having that bench of data science and math folks is pretty unique,” Beaver said.
Having some of the fastest computers in the world doesn’t hurt, either. It’s computing muscle like Titan (and soon Summit) that allows Beaver’s group to train the models that are later deployed on smaller systems.
Such efforts extend current capabilities to field analytics on devices like network sensors to include more complex analytics on a broader set of systems, such as connected/autonomous vehicles and the electric grid, where they will play a critical role in ensuring America’s safety and energy security.
This same combination of computing, data and domain science expertise also allows researchers in the Geographic Information Science and Technology Group to extend the laboratory’s impact to the far reaches of the globe.
GIST researchers model a wide range of phenomena, from population dynamics to the electric grid to disaster management. Such research requires processing a plethora of satellite imagery and other geographic data, work capable of bogging down even the most experienced research team. By harnessing the power of AI, however, researchers accomplish the work with greater speed, accuracy and real-world impact.
For example, GIST researchers have been assisting the Bill and Melinda Gates Foundation in mapping human settlements in Nigeria, some of which were previously unknown, to improve polio vaccination regimes.
Using machine learning, the team analyzed thousands of satellite images to provide the Gates Foundation and the Nigerian government with information on where these remote settlements are located, giving teams on the ground a much better idea of where to go and how much vaccine to carry.
“We are trying to calculate populations based on structures,” said Budhu Bhaduri, GIST group leader and director of ORNL’s Urban Dynamics Institute. “This means processing pixels and identifying patterns in the data, and AI is very efficient at these sorts of tasks.”
The effort, however, is still dependent on humans’ labeling of the data.
“Humans don’t need to see millions of images of a cat to know what a cat is, but a machine does,” Bhaduri said. “The same goes for roads; the challenge is not to find roads, but to define what a road is, how long, how wide, etc.”
Going forward, the group is looking to AI to self-label the data, a feat that would free staff from hours of grunt work and further AI’s role in helping researchers across the scientific spectrum, including in domains such as health care.
ORNL assists numerous agencies, such as the National Cancer Institute, in combing through treasure troves of data to improve diagnoses, treatments and outcomes for wide swaths of the American public, from children to veterans.
In fact, Tourassi’s work with NCI helped ORNL take home trade publication HPCwire’s “Best Use of AI” award at the International Conference for High Performance Computing, Networking, Storage and Analysis in November 2017.
The award recognized ORNL’s contribution to the CANcer Distributed Learning Environment—or CANDLE—project, a DOE and NCI collaboration in which researchers use deep learning to extract information from cancer surveillance reports. Such analyses can locate previously undiscovered relationships within the vast data stores collected by NCI and improve health care for millions.
“AI has applications in health care from bench to bedside,” said Tourassi, “from fundamental research to health care delivery. And we are only getting started.”
The future of AI
Of course, nothing revolutionary is easy, and for all its potential AI still must clear a host of hurdles before it can revolutionize scientific discovery.
Besides the most obvious hurdles of explainability and the mounting petabytes of unlabeled data, there are deeper, more complex issues.
“These networks must be efficient in terms of time,” Bhaduri said. “Decisions are time-critical, and the machine must not only make the correct decision, but it must also do it faster than humans are capable of.”
Adds Potok: “The number of people who are applying AI to problems of science and national security is small. We need smart, talented people to help propel the field forward.”
Researchers are optimistic, however, as the obstacles presented by AI are dwarfed only by its potential to revolutionize our understanding of the world. And while no one can predict the future, some trends are emerging.
“Some successes will be plainly visible,” Womble said. “But perhaps the biggest, most profound impacts will be ironically less visible. Advanced manufacturing will lead to less expensive, more durable products, for instance, including cars that last longer, computers that run faster and houses that are better insulated. You name it, it will get better.”
And scientists will have perhaps the greatest tool for discovery known to man right at their fingertips.
“Rather than conducting costly simulations and experiments, and iterating back and forth, I might just tell the machine, ‘I need to build a superconducting material’ and have it guide the path to discovery,” Potok said. “This capability will allow researchers to extend theories and advance their domains faster than ever thought possible.”
It’s a theme that re-emerges frequently: AI, rather than a threat, is a resource for researchers already at the cutting edge of their fields, particularly at a laboratory perfectly suited for AI innovation.
“AI has enormous potential to revolutionize our understanding of the world,” Nichols said. “Fortunately, ORNL likewise has enormous potential to be a key player in the research and development of AI in the years ahead, and my guess is that today’s big science challenges will become exponentially smaller.”
See also:
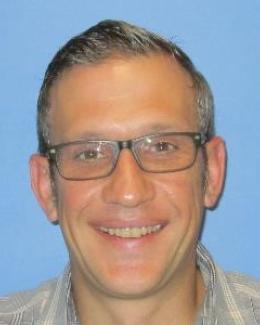