The hazy image shows how satellite imagery looks when first received by the analysts. After processing, the dehazed image is clearer for a scientist to review. Credit: Vandy Tombs/ORNL, U.S. Dept of Energy
Researchers and analysts at the Department of Energy’s Oak Ridge National Laboratory are combining geospatial science and artificial intelligence to reduce the risks posed by nuclear materials while further enabling the peaceful use of nuclear technologies. This new generation of technologies integrates and translates several of ORNL’s core science capabilities into real-world solutions for international partners, such as the International Atomic Energy Agency, or IAEA.
The IAEA deploys inspectors to nuclear facilities around the world to ensure nuclear materials are used safely and securely for peaceful purposes such as advanced energy, agricultural and medical systems. As IAEA inspectors are thinly spread across an increasing number of locations, the agency needs scalable inspection and safeguarding methods.
“Trying to find ways to do things better with automation and geospatial information is a hot topic of interest for the IAEA,” said Scott Stewart, a data scientist for the Nuclear Nonproliferation Division, or NND, at ORNL.
Stewart had attended a conference where scientists from around the world discussed the use of new satellite imaging and machine learning techniques to support IAEA nonproliferation goals remotely.
The IAEA already uses satellite imagery to aid in their safeguards’ verification activities, and with thousands of nuclear facilities around the world, “they're especially interested in seeing machine learning applied to this area, where you've often got so much data that it's too difficult to look through manually,” Stewart said.
While artificial intelligence approaches like machine learning may offer new means to manage huge amounts of geospatial data, implementation is complex and requires unique capabilities.
Training smart systems
ORNL is a national leader in applying AI and machine learning to geospatial science. ORNL researchers’ expertise in these areas helped lab earn an award for the best DOE geospatial science program in November 2022.
Vandy Tombs is an applied mathematician in the lab’s Geographic Data Science Section who joined Stewart at the IAEA conference in Vienna. She uses AI and machine learning to optimize data analysis from geospatial systems. According to Tombs, finding viable training methods is a central challenge for geospatial AI systems.
Much like humans, machine learning systems need training before they can do a job on their own. When analyzing geospatial images, the system trains on a database of images with important features a human has already labeled. Models trained on labeled data currently require more information than a human would to make similar predictions. It can be extremely difficult for systems to accurately classify objects in an image without large databases of labelled information.
The challenge, Tombs said, is that the extremely small features in satellite data make manual labelling a complex task that stretches the limits of contemporary AI.
“Satellites are constantly collecting imagery, so there is actually a lot of data in theory, but the problem is it’s extremely hard to label,” Tombs said. “For example, Maxar satellite images tend to be 16 kilometers by 16 kilometers, so something like a car will be about one or two pixels.” The relatively tiny size of objects in these images makes it difficult for algorithms or human operators to consistently differentiate and accurately label them.
Enhancing computer vision
Researchers in the GeoAI group worked with colleagues across the ORNL Geospatial Science and Human Securities Division to develop a series of improvements to computers’ ability to detect and identify objects in an image. These improvements promise broad applications in areas such as nonproliferation, population modeling and disaster response.
Using machine learning approaches, the GSHSD team created tools for mitigating the effects of haze in urban areas and for detecting clouds. These changes represent a substantial improvement because more than half of the globe is covered by clouds at a given time, rendering large portions of geospatial data unusable by traditional analysis. The new model gives analysts significantly more information from the same dataset by detecting clouds on a pixel-by-pixel basis instead of ignoring images containing a percentage of cloud cover above an arbitrary threshold.
The team has also developed tools for identifying changes in architecture, including damaged buildings and newly built structures. They can also classify structures according to their function, such as residential apartments or industrial buildings.
While many of these programs are extremely accurate, they are not infallible. To account for this, the GeoAI group plans to incorporate tools for quantifying uncertainty in outputs into their model, as well as a querying system.
“For example, in an object-counting use case, an analyst may ask the system ‘How many buildings are in this scene?’” Tombs said. “The responses can be provided with uncertainty estimations and other visualizations to increase trustworthiness in the system.”
Tombs and colleagues in the field have also been investigating methods to train AI systems more efficiently in cases where labeled data is scarce, which is often the case in nuclear nonproliferation. Through a method called transfer learning, researchers train AI systems on datasets that differ slightly from the target dataset but still allow the model to recognize features in the target images. Using transfer learning, researchers can dramatically reduce the amount of labelled training data necessary to create useful AI image recognition models. These techniques, combined with advances in computer hardware, can open the door to a wide range of applications in geospatial computing.
“As computing power gets better, it’s made it a lot easier to bring in techniques from other domains,” said Tombs. “It's going to have more applications in the geospatial domain, which is going to have a ripple effect into nuclear nonproliferation.”
Solving real-world challenges
International nuclear security organizations are seeking to use new geospatial technologies in a broad range of applications. The integration of these technologies is already having a significant effect, according to Jeremy Patterson, ORNL’s deputy program manager for support to the National Nuclear Security Administration’s Office of Nuclear Smuggling Detection and Deterrence, or NSDD. NSDD partners with more than 80 countries to build counter nuclear smuggling capacity at official ports of entry such as airports and seaports as well as frontier borders and partner country interiors.
“Over the last two years, we have started supporting the NSDD program with satellite imagery and remote sensing capabilities from GSHSD, and it's turning into a pretty successful collaboration,” Patterson said. Patterson and his colleague Mike Channer co-lead ORNL’s GeoNonPro team, a collaboration between NND and GSHSD to leverage commercial satellite and remote sensing capabilities for nonproliferation missions.
NSDD has recently begun using satellite and remote sensing data to determine the best locations for building their capacity to counter nuclear smuggling through placement of equipment like cameras and radiation detectors. As AI continues to increase the efficacy of geospatial imagery, collaborative efforts between researchers and groups like NSDD are creating new nonproliferation use cases.
Analysts like Patterson are particularly interested in computer-based tracking and modeling of building construction and radioactive material movement through periodic satellite imaging. Safeguards inspectors could access updated information faster as machine learning algorithms deliver relevant information from large datasets more quickly than manual processes.
Looking to the future, Patterson is confident geospatial science will continue to positively affect nuclear security approaches and outcomes, especially as new commercial capabilities continue to be released.
“There's just so much satellite and remote sensing capability that exists now, and that's going to continue to grow exponentially,” he said.
In addition to imagery data expansions, innovations in computer science and geospatial imaging promise new innovations in the years to come.
“We’ll keep unlocking new use cases as the number of different types of sensors that are being put on satellites for Earth observation grows,” Patterson said. “We'll be able to apply innovative techniques to make that data digestible and usable for actual mission applications.”
UT-Battelle manages ORNL for the Department of Energy’s Office of Science, the single largest supporter of basic research in the physical sciences in the United States. The Office of Science is working to address some of the most pressing challenges of our time. For more information, please visit energy.gov/science. – Galen Fader
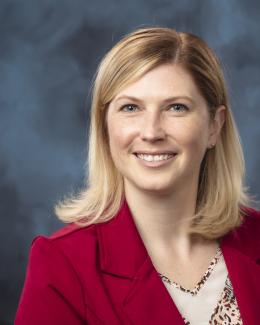