Topics:
Scientific Achievement
Developed a deep-learning approach to automatically create libraries of structural and electronic properties of atomic defects in 2D materials.
Significance and Impact
The properties and functionalities of materials are intrinsically linked to the presence, properties, and populations of structural defects, and knowing them is key to designing and developing stronger and better materials for information and energy applications.
Research Details
- Dynamic scanning transmission electron microscopy (STEM) was used to create multiple non-equilibrium atomic configurations of Si impurities in graphene.
- An automated machine learning method was developed to extract and classify defects from dynamic STEM data.
- Density functional theory provided electronic properties that are verified with atomically resolved scanning tunneling microscopy images
M. Ziatdinov, O. Dyck, X. Li, B. G. Sumpter, S. Jesse, R. K. Vasudevan, and S. V. Kalinin, Building and exploring libraries of atomic defects in graphene: Scanning transmission electron and scanning tunneling microscopy study, Sc. Adv. 5, eaaw8989 (2019).
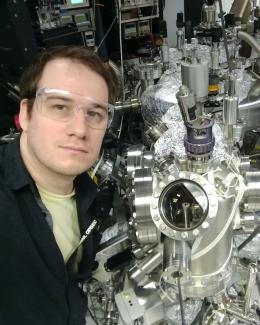