Topics:
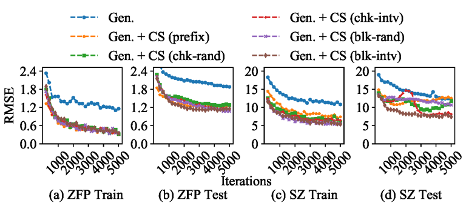
This graphic illustrates the compression ratio regression of the two compressors in different samplings.
The Science
A team of ORNL researchers built a deep neural network to estimate the compressibility of scientific data and show that adding compressor-specific features can greatly improve the performance of prediction. To achieve this, the researchers:
- extracted features from the data characteristics or the inner mechanisms of compressors;
- compared the performance of compressor-specific features under different samplings; and
- compared the performance of deep learning with the sampling and analytical methods.
The Impact
The novel neural network:
- represents a keystone component that will enable the best uses of standard compressors on scientific data and allow researchers to better manage massive data sets.
- performs better than the previous standard of predicting data compressibility (using biased estimation and a white-box analytical model).
PI(s)/Facility Lead(s): Scott Klasky (ORNL)
Publication: Qin, Zhenlu, et al. Estimating Lossy Compressibility of Scientific Data Using Deep Neural Networks. IEEE Letters of the Computer Society 3.1 (2020): 5-8. DOI: https://doi.org/10.1109/LOCS.2020.2971940.Facility: Work was performed at Oak Ridge National Laboratory
Funding: DOE ASCR
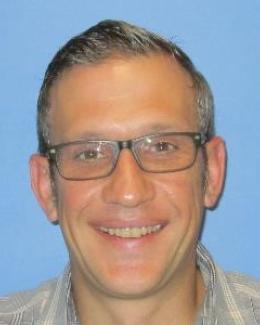