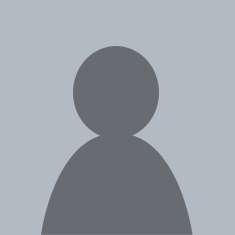
Bio
Subrata Mukherjee graduated from Michigan State University in 2023 with a PhD in Electrical Engineering. After completing his PhD, he served as a Postdoctoral Fellow in the Division of Imaging, Diagnostics, and Software Reliability at the US Food and Drug Administration until July 2024. Currently, he is a Postdoctoral Research Associate at Oak Ridge National Laboratory, a position he has held since July 2024.
Subrata has research and industrial experience focused on algorithm development, non-invasive imaging, non-destructive evaluation, data analysis, signal and image processing, machine learning, statistical inference, and fault diagnostics. At ORNL, his research focuses on sensors and automation in complex real-world environments. This includes developing specialized algorithms and software tools for sensor management, fault detection and correction, modeling, optimization, and applying Machine Learning (ML) and Artificial Intelligence (AI) to enhance fault diagnostics, process monitoring, and advanced control methods. These sophisticated data-driven and physics-based models and tools have applications across various fields, including electrical grid monitoring, smart manufacturing, water treatment, desalination, and other non-destructive monitoring applications.
A terse description of his research activities is available here .
Professional Experience
Postdoctoral Research Associate - Oak Ridge National Laboratory
Sensors and Embedded Systems Group [July 2024 – Present]
At ORNL, he is working in the Sensors and Embedded Systems Group of the Electrification and Energy Infrastructures Division (EEID). His primary responsibility involves the development and application of Machine Learning (ML) and Artificial Intelligence (AI) algorithms to enhance fault diagnostics, process monitoring, and advanced control methods. These sophisticated data-driven and physics-based dynamic models find application across diverse fields such as electrical grid monitoring, smart manufacturing, water treatment, and desalination.
Postdoctoral Fellow - US Food and Drug Administration
Division of Imaging, Diagnostics, and Software Reliability [August 2023 – July 2024]
At FDA, he worked on the project “Prediction of Response to Therapy for Metastatic Breast Cancer: Joint Analysis of Radiologic and Genomic Data Using Machine Learning”. He developed an image registration-based automated lesion correspondence and matching (RAMAC) algorithm and designed it into a regulatory science tool (RST). This algorithm dynamically tracks both target and non-target entities, addressing the variability across different timepoints and radiologists in longitudinal data analysis. He developed joint modeling of the longitudinal and time-to-event data for metastatic breast cancer (mBC) progression risk prediction, performing lesion and organ segmentation using deep learning frameworks. He also actively participated in reviewing AI/ML consults related to medical imaging devices and diagnostics and attended regulatory discussion meetings at the FDA.
Research Associate - Michigan State University
Department of Electrical and Computer Engineering [August 2018 – July 2023]
During his PhD at MSU, he worked as a research assistant (RA) where he developed robust automated defect detection and identification algorithms using data collected from novel near-field electromagnetic sensors. He led various projects funded by industrial partners such as Electric Power Research Institute (EPRI), Gas Technology Institute (GTI), and other DOT, DOE-based PHMSA projects. His responsibilities included developing flexible deep learning frameworks, spatially adaptive denoising algorithms, efficient data augmentation, digital twinning and fusion schemes, sophisticated data compression algorithms, defect tracking algorithms, and nondestructive sensor development for inline inspection (ILI).
Advanced Algorithm Researcher - Analog Garage
[May 2022 – September 2022]
At Analog Garage, he worked on the Advanced Battery Monitoring (ABM) project, where he developed novel statistics-based transfer learning algorithms for electric vehicle battery state of health (SOH) estimation across various battery types. Additionally, he developed synthetic battery aging models in Ansys following complex test protocols similar to real experiments.
Solution Integrator - Ericsson
[September 2015 – May 2018]
He worked in R&D and operations for Workforce Management (WFM) at Ericsson in Kolkata, India. He developed optimized algorithms and new customized routines and policies for mobile touch customization using C#, Click, and JQuery.
Awards
Recipient of the 2018 Engineering Distinguished Scholar Award at Michigan State University.
Education
Doctor of Philosophy (Ph.D.), Electrical and Computer Engineering: Michigan State University, 2023
Dissertation: Machine learning based efficient automated NDE methods for defect diagnostics
- Bachelor of Technology (B. Tech) in Electronics & Communication Engineering, 2015
Professional Service
Reviewer: IEEE Sensors, IEEE Transaction on Instrumentation and Measurement, Springer Nature-Journal of Nondestructive Evaluation (JNDE), Research in Nondestructive Evaluation (RNDE), IEEE PHM, IMECE (ASME), British Journal of Radiology (BJR), and Medical Physics
Other Publications
- Improved joint modelling of radiomic features and hazard by image registration aided longitudinal CT data,
Subrata Mukherjee, Qian Cao, Thibaud Coroller, Craig Wang (submitted in IOP Science) - Robust defect detection under uncertainties using spatially adaptive Capacitive Sensing, Subrata Mukherjee, Lalita Udpa, Yiming Deng, Journal of Applied Physics 131, 234901 (2022).
- Dynamic Defect Detection in Fast, Robust NDE Methods by Transfer Learning Based Optimally Binned Hypothesis Tests, Subrata Mukherjee, Lalita Udpa, Yiming Deng Research in Nondestructive Evaluation.
- Enhanced Defect Detection in NDE Using Registration aided Heterogeneous Data Fusion, Subrata Mukherjee, Ciaron Hamilton, Xuhui Huang, Lalita Udpa, Yiming Deng, NDT & E International, 102964
- Inline Pipeline Inspection Using Hybrid Deep Learning Aided Endoscopic Laser Profiling, Subrata Mukherjee, Yiming Deng, Journal of Non-destructive Evaluation, Springer (2022)
- A Kriging-Based Magnetic Flux Leakage Method for Fast Defect Detection in Massive Pipelines, Subrata Mukherjee, Xuhui Huang, Lalita Udpa, Yiming Deng. ASME Journal of Nondestructive Evaluation, Diagnostics and Prognostics of Engineering Systems, 2021.
- Navigation Algorithm Based on The Boundary Line of Tillage Soil Combined with Guided Filtering and Improved Anti-Noise Morphology, Wei Lu, Mengie Zeng, Ling Wang, Hui Luo, Subrata Mukherjee, Xuhui Huang, Yiming Deng. Sensors (Basel), 19(18):3918, 2019.
- Adaptive Segmentation-based Evaluation of Material Properties of Dielectric Sheets using Microwave NDE, Shankar Aenagandula, Subrata Mukherjee, Neeraj Rao, Yiming Deng. Nondestructive Testing, July 2023.
- Fast VGG: an advanced pre-trained deep learning framework for multi-layered composite NDE via multifrequency near-field microwave imaging
- Deep learning-assisted structural health monitoring: acoustic emission analysis and domain adaptation with intelligent fiber optic signal processing
- Image Registration based Automated Lesion Correspondence and Tracking Pipeline for Longitudinal CT Data, Subrata Mukherjee, Thibaud Coroller, Craig Wang, Ravi k. Samala, Berkman Sahiner, Nicholas Petrick, Qian Cao.
- Early Assessment of Progression-Free Survival in Metastatic Breast Cancer: Radiomic Analysis from Initial Post-Treatment CT Data, Subrata Mukherjee, Qian Cao, Thibaud Coroller, Craig Wang, RSNA 2024 (accepted).
- Defect Tracking Via NDE Based Transfer Learning, Subrata Mukherjee, Vivek Rathod, Xuhui Huang, Lalita Udpa, Yiming Deng, IEEE PHM Detroit (ICPHM) 2020.
- Accurate Material Characterization of Wideband RF Signals via Registration-based Curve Fitting Model using Microstrip Transmission Line, Subrata Mukherjee, Lalita Udpa, Yiming Deng, accepted in IEEE PHM Quebec (ICPHM) 2023.
- NDE Based Cost-Effective Detection of Obtrusive and Coincident Defects in Pipelines Under Uncertainties, Subrata Mukherjee, Xuhui Huang, Lalita Udpa, Yiming Deng, IEEE PHM Paris 2019.
- A Kriging Based Fast and Efficient Method for Defect Detection in Massive Pipelines Using Magnetic Flux Leakages, Subrata Mukherjee, Xuhui Huang, Lalita Udpa, Yiming Deng, IMECE 2020.
- Extracting Mode Converted Guided Wave Response Due to Delamination Using Embedded Thin Film Sensors, Vivek Rathod, Subrata Mukherjee, Lalita Udpa, Yiming Deng, IEEE PHM Detroit (ICPHM) 2020.
- Machine Learning Enabled Damage Classification in Composite Laminated Beams Using Mode Conversion Quantification, Vivek Rathod, Subrata Mukherjee, Yiming Deng, SPIE Proceedings 2020.
- Fatigue Damage Prognosis in Adhesive Bonded Composite Lap-Joints Using Guided Waves, Rajendra P Palanisamy, Subrata Mukherjee, Lalita Udpa, Yiming Deng, IEEE PHM Detroit (ICPHM) 2020.
- Rapid Material Characterization Using Smart Skin with Functional Data Analysis, Rajendra P Palanisamy, Subrata Mukherjee, Mahmood, Yiming Deng, IEEE PHM Europe (PHME) 2021.
- FEM of Magnetic Flux Leakage Signal for Uncertainty Estimation in Crack Depth Classification using Bayesian Convolutional Neural Network and Deep Ensemble.
- Polymer Materials Characterization Using a Novel C-Shaped Dual-Band Transmission Based Differential Sensing