Philipe Ambrozio Dias. Credit: Genevieve Martin/ORNL, U.S. Dept. of Energy
Having lived on three continents spanning the world’s four hemispheres, Philipe Ambrozio Dias understands the difficulties of moving to a new place. Dias is a native of Brazil, but his academic career took him to Germany, Italy, and eventually the United States, where he researches climate change’s impact on human geography and national security.
Dias, a research and development associate in computer vision and machine learning at Department of Energy’s Oak Ridge National Laboratory, works to improve the speed at which machine learning models can be adapted to a new challenge. He and his colleagues build machine learning algorithms to detect structures of interest and patterns of change around the planet — whether from war, weather, or other major incidents — based on images collected from remote sensing platforms. The information derived from these models can be used for population mapping and land characterization or to reveal patterns on how people rebuild or relocate based on the need for stable access to food, water or adequate shelter.
“I have a personal connection to understanding how climate may force migration,” said Dias. “Moving always comes with a burden, so trying to mitigate those challenges or improve planning can impact cascading issues at borders or with international relationships.”
As the Earth changes, governments need information to make decisions. Images from around the world are collected each day and scanned by algorithms to identify buildings, agricultural space, landmarks, and other features. Each algorithm has specific parameters for identifying features based on the need of the user.
Dias’ work focuses on algorithms of the future that can be easily adapted to a variety of scenarios and are trained faster in a data-efficient and less human interaction. He develops large models used across tasks to integrate speed and accuracy into the results. When a natural disaster happens, for example, and emergency response teams need to know where to send resources for search and rescue, better algorithms can provide accurate information to get vital emergency supplies to communities faster.
Starting in graduate school, Dias has applied machine learning-based image processing to wastewater treatment, agriculture, assisted living for healthcare scenarios, and now human security. With knowledge in these different domains and techniques, he anticipates the needs and challenges of researchers and can make better image-processing algorithms.
Dias found purpose applying his knowledge to national security research, attributing the discovery to his experience moving from country to country in pursuit of his doctorate. He recognized that with moving came challenges such as learning a new language and culture — even learning how to dress for the weather. Though his moves were by choice, he understands the added hardships on individuals and communities forced to migrate. “People are often forced to move because of different circumstances,” he said. “With the right mechanisms in place, it could make this process less traumatic.”
When asked about his future research, Dias quickly highlights climate change and the dire need for science to inform how people live and move. Changes to food availability, weather patterns, wildfires and geopolitics impact the environment as much as governments and societies. “There is no bigger problem to be working on in the near future.”
UT-Battelle manages ORNL for the Department of Energy’s Office of Science, the single largest supporter of basic research in the physical sciences in the United States. The Office of Science is working to address some of the most pressing challenges of our time. For more information, please visit energy.gov/science. — Liz Neunsinger
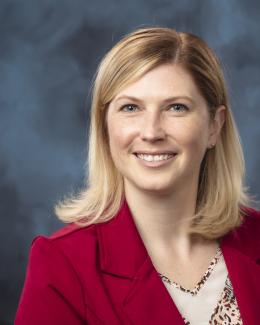