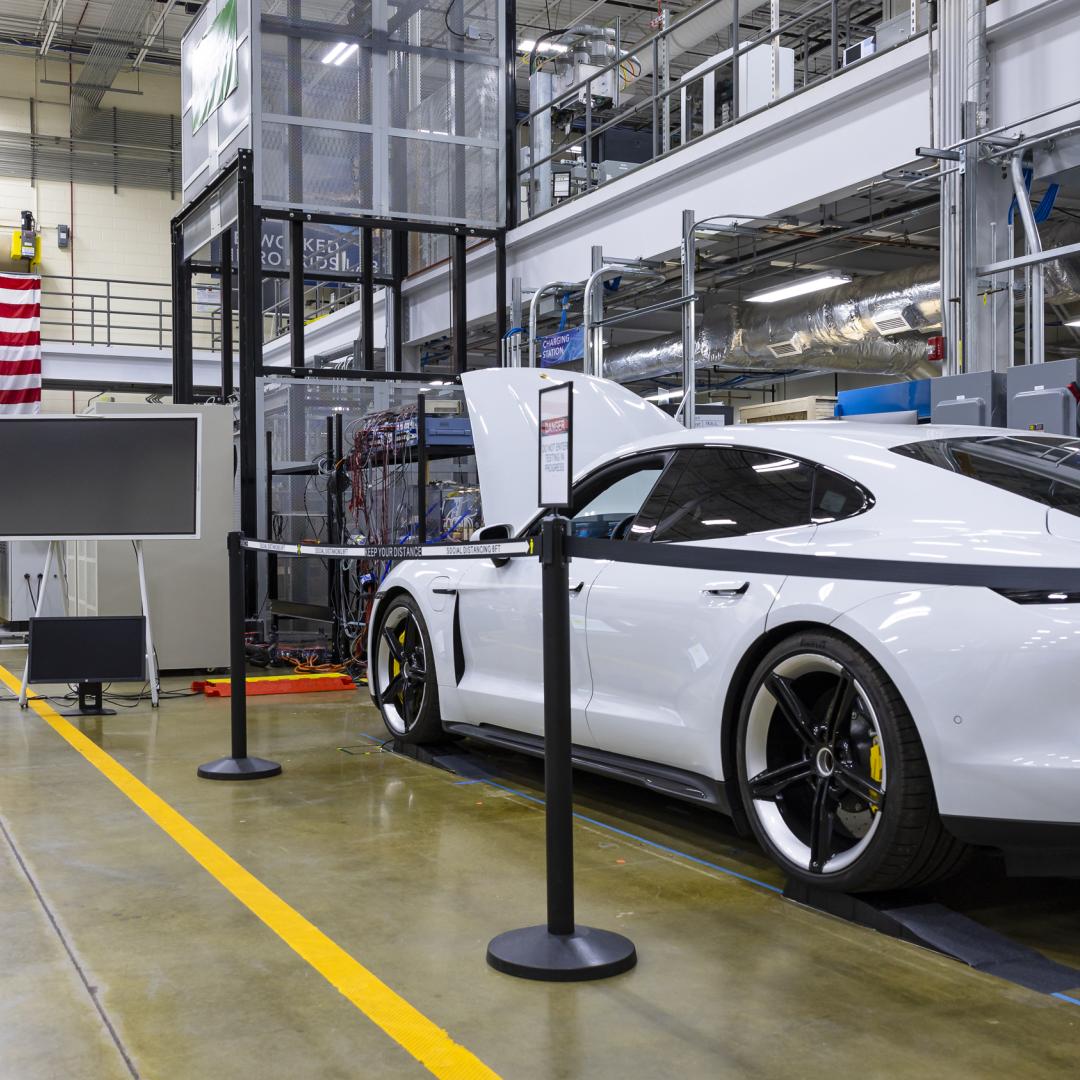
Filter News
Area of Research
- Advanced Manufacturing (18)
- Biology and Environment (17)
- Building Technologies (1)
- Clean Energy (64)
- Computational Engineering (1)
- Computer Science (9)
- Fusion Energy (1)
- Materials (41)
- Materials for Computing (9)
- National Security (9)
- Neutron Science (10)
- Nuclear Science and Technology (2)
- Quantum information Science (4)
- Renewable Energy (1)
- Supercomputing (34)
News Type
News Topics
- (-) 3-D Printing/Advanced Manufacturing (75)
- (-) Artificial Intelligence (42)
- (-) Big Data (24)
- (-) Hydropower (6)
- (-) Machine Learning (23)
- (-) Polymers (21)
- (-) Quantum Science (36)
- Advanced Reactors (23)
- Bioenergy (39)
- Biology (39)
- Biomedical (28)
- Biotechnology (10)
- Buildings (32)
- Chemical Sciences (38)
- Clean Water (14)
- Climate Change (44)
- Composites (18)
- Computer Science (96)
- Coronavirus (28)
- Critical Materials (23)
- Cybersecurity (20)
- Decarbonization (27)
- Education (3)
- Element Discovery (1)
- Energy Storage (72)
- Environment (79)
- Exascale Computing (10)
- Fossil Energy (1)
- Frontier (15)
- Fusion (23)
- Grid (35)
- High-Performance Computing (37)
- Irradiation (2)
- Isotopes (22)
- ITER (5)
- Materials (94)
- Materials Science (83)
- Mathematics (1)
- Mercury (5)
- Microelectronics (1)
- Microscopy (27)
- Molten Salt (7)
- Nanotechnology (38)
- National Security (21)
- Net Zero (4)
- Neutron Science (76)
- Nuclear Energy (45)
- Partnerships (28)
- Physics (28)
- Quantum Computing (13)
- Renewable Energy (1)
- Security (12)
- Simulation (15)
- Space Exploration (13)
- Statistics (3)
- Summit (26)
- Sustainable Energy (75)
- Transformational Challenge Reactor (4)
- Transportation (60)
Media Contacts
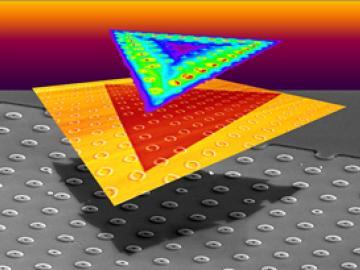
A team led by scientists at the Department of Energy’s Oak Ridge National Laboratory explored how atomically thin two-dimensional (2D) crystals can grow over 3D objects and how the curvature of those objects can stretch and strain the
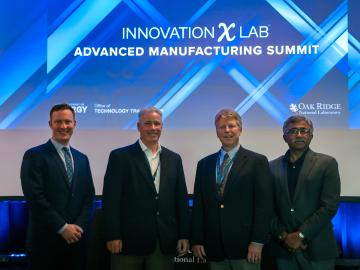
OAK RIDGE, Tenn., May 8, 2019—Oak Ridge National Laboratory and Lincoln Electric (NASDAQ: LECO) announced their continued collaboration on large-scale, robotic additive manufacturing technology at the Department of Energy’s Advanced Manufacturing InnovationXLab Summit.
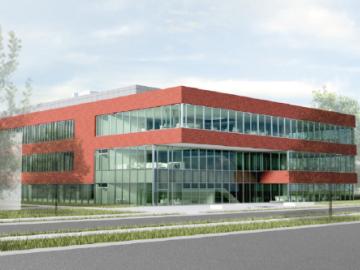
OAK RIDGE, Tenn., May 7, 2019—Energy Secretary Rick Perry, Congressman Chuck Fleischmann and lab officials today broke ground on a multipurpose research facility that will provide state-of-the-art laboratory space
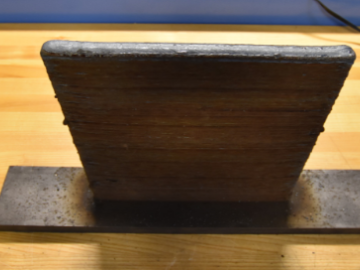
A novel additive manufacturing method developed by researchers at Oak Ridge National Laboratory could be a promising alternative for low-cost, high-quality production of large-scale metal parts with less material waste.

Oak Ridge National Laboratory is using artificial intelligence to analyze data from published medical studies associated with bullying to reveal the potential of broader impacts, such as mental illness or disease.
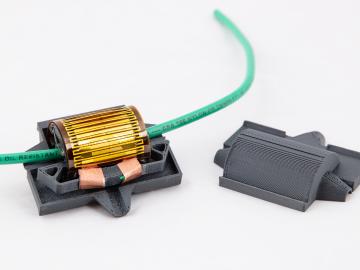
Scientists at Oak Ridge National Laboratory have developed a low-cost, printed, flexible sensor that can wrap around power cables to precisely monitor electrical loads from household appliances to support grid operations.
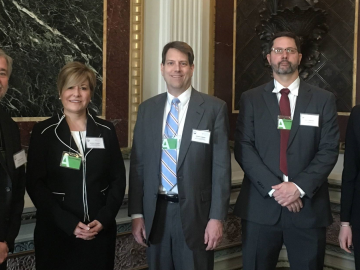
OAK RIDGE, Tenn., March 4, 2019—A team of researchers from the Department of Energy’s Oak Ridge National Laboratory Health Data Sciences Institute have harnessed the power of artificial intelligence to better match cancer patients with clinical trials.
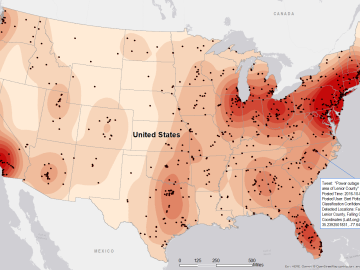
Gleaning valuable data from social platforms such as Twitter—particularly to map out critical location information during emergencies— has become more effective and efficient thanks to Oak Ridge National Laboratory.
OAK RIDGE, Tenn., Feb. 12, 2019—A team of researchers from the Department of Energy’s Oak Ridge and Los Alamos National Laboratories has partnered with EPB, a Chattanooga utility and telecommunications company, to demonstrate the effectiveness of metro-scale quantum key distribution (QKD).
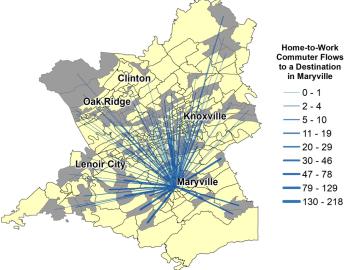
Oak Ridge National Laboratory geospatial scientists who study the movement of people are using advanced machine learning methods to better predict home-to-work commuting patterns.