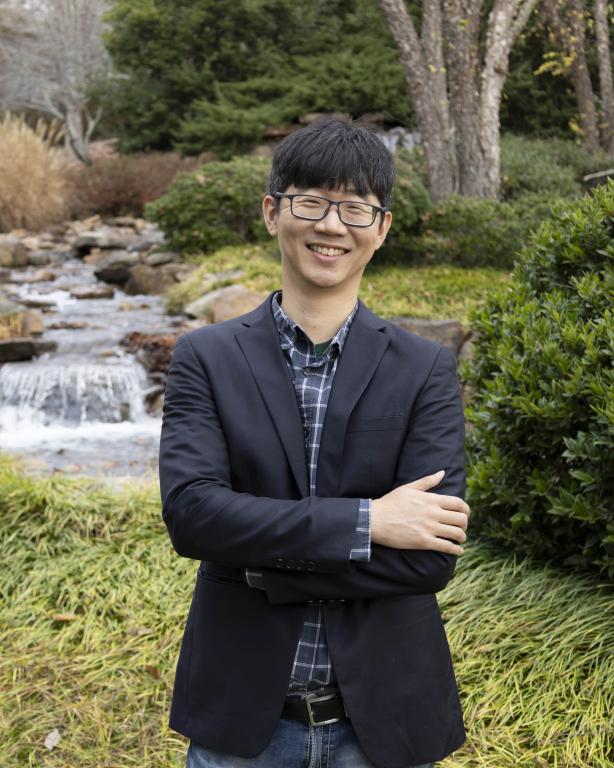
Sangkeun “Matt” Lee, a computer scientist in the Discrete Algorithms Group, received the Best Poster Award at the Institute of Electrical and Electronics Engineers 24th International Conference on Information Reuse and Integration.
Lee’s paper at the August conference in Bellevue, Washington, combined weather and power outage data for three states – Texas, Michigan and Hawaii – and used a machine learning model to predict how extreme weather such as thunderstorms, floods and tornadoes would affect local power grids and to estimate the risk for outages. The paper relied on data from the National Weather Service and the U.S. Department of Energy’s Environment for Analysis of Geo-Located Energy Information, or EAGLE-I, database.
EAGLE-I, maintained by ORNL, collects outage data every 15 minutes from utility providers serving more than 140 million customers nationwide. Emergency management agencies and first responders routinely rely on the database for an instant real-time snapshot of situations on the ground during disasters.
“Predicting risks during these extreme situations will become increasingly important as climate change leads to rare weather events such as floods or tornadoes occurring in regions where these events have been mostly unfamiliar,” Lee said. “We need ways to prioritize emergency response before, during and after these events.”
Lee led the presentation as part of a team with ORNL colleagues Gang Seob Jung, Jong Youl Choi, Anika Tabassum, Nils Stenvig and Supriya Chinthavali. The team found their models achieved an accuracy rate of better than 90% for predictions in states where enough historical data was available.
“We explained the challenges of preparing and processing these datasets for machine learning, and we highlighted the feasibility of predicting the power outage risk from extreme weather events at the state level,” Lee said. “We still have a long way to go to make this approach practical in the real world. We hope our study serves as a foundation for developing more sophisticated models that can enable better preparedness and response strategies for emergency management.”
Future efforts will focus on zeroing in to predict outage risk at the level of individual counties.
UT-Battelle manages ORNL for DOE’s Office of Science, the single largest supporter of basic research in the physical sciences in the United States. DOE’s Office of Science is working to address some of the most pressing challenges of our time. For more information, visit https://energy.gov/science. - Matt Lakin
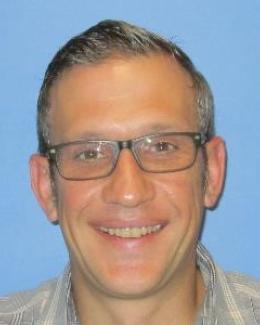