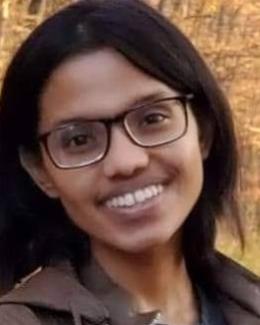
Bio
Anika Tabassum is contributing towards developing deep Learning for multi-scale and multimodal data evolved from energy research. Her research interest broadly lies in robust and scalable domain-guided ML for scientific discovery. She has been selected as RISING STAR 2023 by UT Austin and an outstanding postdoctoral award from her division at ORNL in 2022. She received her Ph.D. from the Department of Computer Science at Virginia Tech where she worked on bringing domain-guided ML to address multiple challenges to prepare and mitigate power system failures and disaster vulnerabilities. Her Ph.D. research work was funded by NSF Urban Computing fellowship. She won 1st prize in designing the COVID-19 forecasting model for the Facebook-CDC challenge. She has published in multiple venues as NeuRIPS, AAAI, ACM SigKDD, CIKM, IEEE BigData, IAAI, and journals like ACM TIST and Elsevier.
Professional Experience
Selected Projects at ORNL:
- Li-ion battery Temperature modeling: Develop a domain generalizable deep-learning model to model thermal runaway for various conditions of Li-ion battery properties.
Collaborator: Stevens Institute of Technology December 2022- August 2023
Deep learning Models: LSTM, Transformer, Meta-learning.
- Reduced order Representation for neuromorphic chip simulator: Develop a network-based sequential model to predict the state of neurons.
Collaborator: Sandia National Lab April 2023 - Present
Deep learning models: GNN, Transformer.
- ML Data reduction architecture for Fusion Plasma Simulator: Develop a data reduction technique for identifying plasma particle distributions using XGC gyrokinetic simulation data.
Collaborator: Princeton Plasma Physics Lab June 2022 - December 2022
Deep learning models: ResNet, Hypernetworks, Vision Transformer, Variational AutoEncoder
Awards
- Rising Stars in Computational & Data Sciences, University of Texas Austin, 2023.
- Outstanding Postdoc researcher award in Computer Science and Mathematics Division (CSMD), 2022.
- Facebook COVID-19 Symptom Data Challenge, 1st prize (Team DEEP OUTBREAK), 2020.
- NSF Fellowship, 2019-21.
- Travel Award CIKM, 2021.
- Travel award SIGKDD, 2019 & 2020.
Education
- PhD: Computer Science, Virginia Tech
- BSc. : Computer Science & Engineering, Bangladesh University of Engineering & Technology, Bangladesh
Professional Service
- Program Committee 2024: ICLR 2024, AAAI Social Impact 2024
- Program Committee 2023: NeurIPS 2023, ICML Synergy of Scientific ML and Modeling 2023, ECML PKDD 2023
- Program Committee 2022: IEEE Big Data 2022., ECML PKDD 2023
- Session Chair: ECML PKDD 2021, ECML PKDD 2024
- Organizer: IEEE BigData Tools, Methods, and Use-cases for Innovative Scientific Discovery (BTSD) Workshop 2022.
- Reviewer: ICDM, KDD, WWW, SDM, TKDD, IEEE BigData, ECML PKDD, IEEE TPAMI.
Professional Affiliations
- Association of Computing Machinery (ACM)
- NSF Urban Computing Certificate, Virginia Tech
Specialized Equipment
- Programming: Python, Matlab, Pytorch, C++
- Deep Learning: CNN, ResNet, UNet, variants of LSTM, Transformer, Vision Transformer, NBeats, GAN, GNN, VAE,
- Large-scale training: Multiprocessing, PyPy, Pytorch DDP
- Database: PostgreSQL, SqLite.
- Tools: VSCode, Jupyter Notebook, Google Colab
- Computing: ORNL Summit, CADES, Frontier
Publications
Other Publications
PhD Dissertation: Explainable and Network-based Approaches for Decision-making in Emergency Management, Virginia Tech, 2021.
Selected Workshops:
- Ziyu Lu, Anika Tabassum, Shruti Kulkarni, J. Nathan Kutz, Eric Shea-Brown, Seung-Hwan Lim. Attention for Causal Relationship Discovery from Biological Neural Dynamics. NeuRIPS Causality Representation Learning Workshop. 2023.
- Success and Failure Analysis of Foundational and Few-shot Image Segmentation Models as a Case Study on Microstructure Characterization. Monterey Data Conference, 2023.
- Temperature Modeling through Invariance Learning Representation Ensuring Li-ion Battery Safety. ORNL AI Expo 2023.
- Anika Tabassum, Nikhil Muralidhar, Ramakrishnan Kannan, and Srikanth Allu. Li-ion Battery Material phase prediction through Hierarchical Curriculum Learning. AI for Science Workshop, NeuRIPS 2022.