Filter News
Area of Research
News Type
News Topics
- (-) Hydropower (1)
- (-) Machine Learning (1)
- 3-D Printing/Advanced Manufacturing (13)
- Bioenergy (2)
- Biology (1)
- Biomedical (1)
- Biotechnology (1)
- Buildings (12)
- Chemical Sciences (7)
- Clean Water (1)
- Climate Change (3)
- Composites (5)
- Coronavirus (1)
- Critical Materials (2)
- Cybersecurity (2)
- Decarbonization (14)
- Energy Storage (14)
- Environment (3)
- Fossil Energy (1)
- Grid (12)
- Materials (8)
- Materials Science (2)
- Microelectronics (1)
- Microscopy (1)
- National Security (2)
- Net Zero (1)
- Neutron Science (2)
- Nuclear Energy (1)
- Partnerships (7)
- Polymers (1)
- Renewable Energy (1)
- Security (1)
- Simulation (2)
- Sustainable Energy (5)
- Transportation (12)
Media Contacts
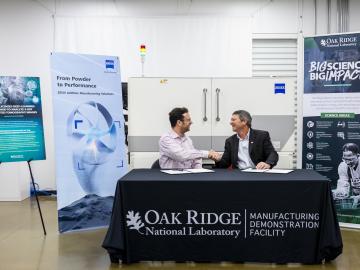
A licensing agreement between the Department of Energy’s Oak Ridge National Laboratory and research partner ZEISS will enable industrial X-ray computed tomography, or CT, to perform rapid evaluations of 3D-printed components using ORNL’s machine

A new report published by ORNL assessed how advanced manufacturing and materials, such as 3D printing and novel component coatings, could offer solutions to modernize the existing fleet and design new approaches to hydropower.