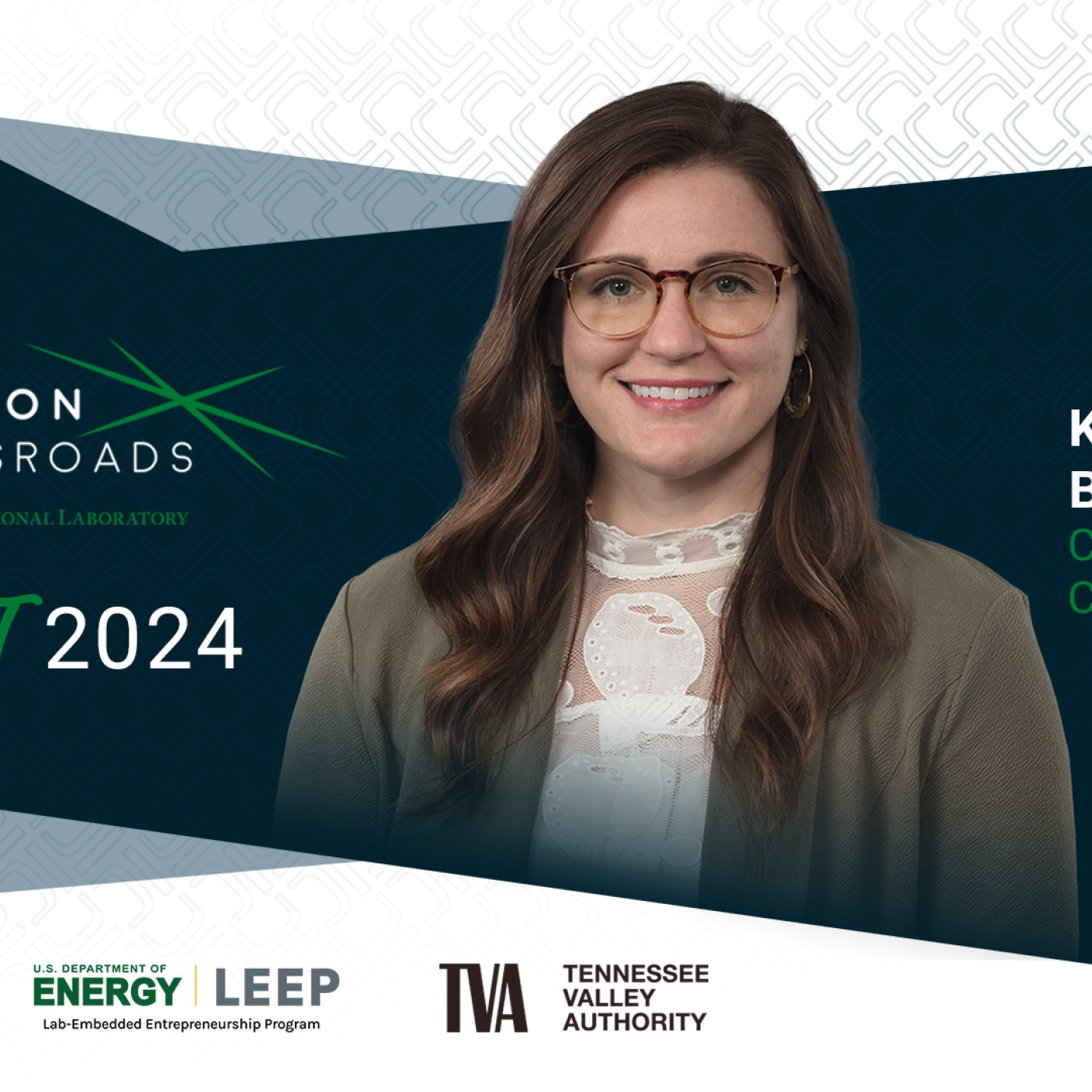
Filter News
Area of Research
News Topics
- (-) Bioenergy (22)
- (-) Clean Water (5)
- (-) Frontier (19)
- (-) Fusion (9)
- (-) Grid (16)
- (-) Machine Learning (15)
- (-) Nanotechnology (7)
- (-) Polymers (4)
- (-) Quantum Science (9)
- (-) Space Exploration (4)
- 3-D Printing/Advanced Manufacturing (20)
- Advanced Reactors (3)
- Artificial Intelligence (26)
- Big Data (10)
- Biology (29)
- Biomedical (7)
- Biotechnology (6)
- Buildings (14)
- Chemical Sciences (24)
- Climate Change (31)
- Composites (6)
- Computer Science (23)
- Coronavirus (4)
- Critical Materials (6)
- Cybersecurity (9)
- Decarbonization (30)
- Education (3)
- Emergency (1)
- Energy Storage (21)
- Environment (43)
- Exascale Computing (15)
- Fossil Energy (2)
- High-Performance Computing (33)
- Hydropower (3)
- Irradiation (2)
- Isotopes (11)
- Materials (59)
- Materials Science (16)
- Mathematics (2)
- Mercury (2)
- Microelectronics (2)
- Microscopy (7)
- Molten Salt (1)
- National Security (21)
- Net Zero (5)
- Neutron Science (32)
- Nuclear Energy (21)
- Partnerships (24)
- Physics (14)
- Quantum Computing (12)
- Renewable Energy (2)
- Security (3)
- Simulation (29)
- Software (1)
- Summit (9)
- Sustainable Energy (17)
- Transportation (18)
Media Contacts
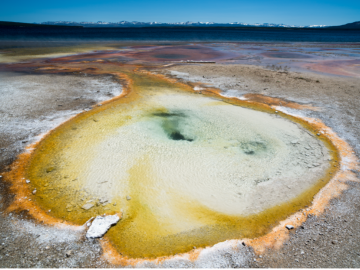
Oak Ridge National Laboratory scientists studied hot springs on different continents and found similarities in how some microbes adapted despite their geographic diversity.
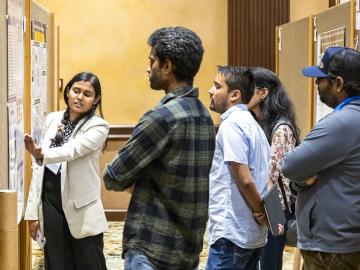
Speakers, scientific workshops, speed networking, a student poster showcase and more energized the Annual User Meeting of the Department of Energy’s Center for Nanophase Materials Sciences, or CNMS, Aug. 7-10, near Market Square in downtown Knoxville, Tennessee.
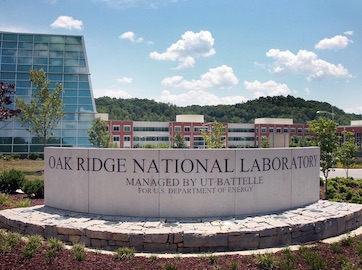
The Department of Energy’s Office of Science has selected three ORNL research teams to receive funding through DOE’s new Biopreparedness Research Virtual Environment initiative.
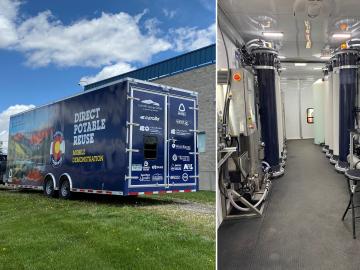
Researchers at ORNL are developing advanced automation techniques for desalination and water treatment plants, enabling them to save energy while providing affordable drinking water to small, parched communities without high-quality water supplies.
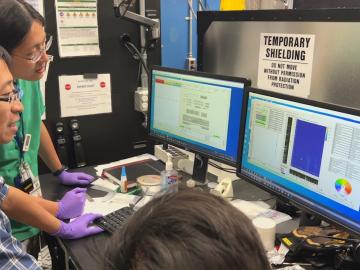
Neutron experiments can take days to complete, requiring researchers to work long shifts to monitor progress and make necessary adjustments. But thanks to advances in artificial intelligence and machine learning, experiments can now be done remotely and in half the time.

Outside the high-performance computing, or HPC, community, exascale may seem more like fodder for science fiction than a powerful tool for scientific research. Yet, when seen through the lens of real-world applications, exascale computing goes from ethereal concept to tangible reality with exceptional benefits.
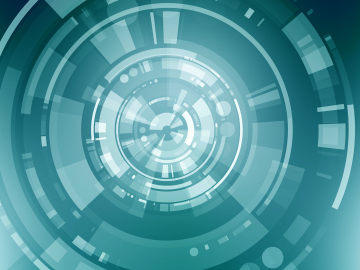
A new nanoscience study led by a researcher at ORNL takes a big-picture look at how scientists study materials at the smallest scales.
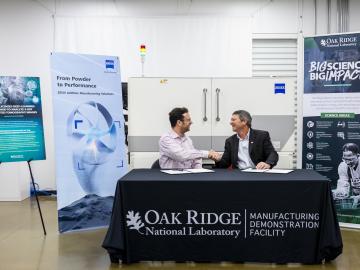
A licensing agreement between the Department of Energy’s Oak Ridge National Laboratory and research partner ZEISS will enable industrial X-ray computed tomography, or CT, to perform rapid evaluations of 3D-printed components using ORNL’s machine
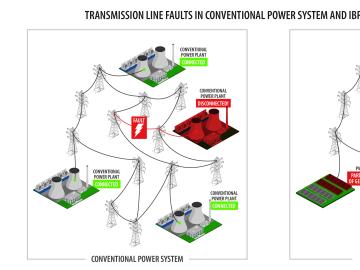
Researchers at the Department of Energy’s Oak Ridge National Laboratory are leading the way in understanding the effects of electrical faults in the modern U.S. power grid.
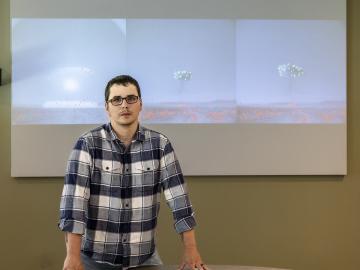
Cody Lloyd became a nuclear engineer because of his interest in the Manhattan Project, the United States’ mission to advance nuclear science to end World War II. As a research associate in nuclear forensics at ORNL, Lloyd now teaches computers to interpret data from imagery of nuclear weapons tests from the 1950s and early 1960s, bringing his childhood fascination into his career