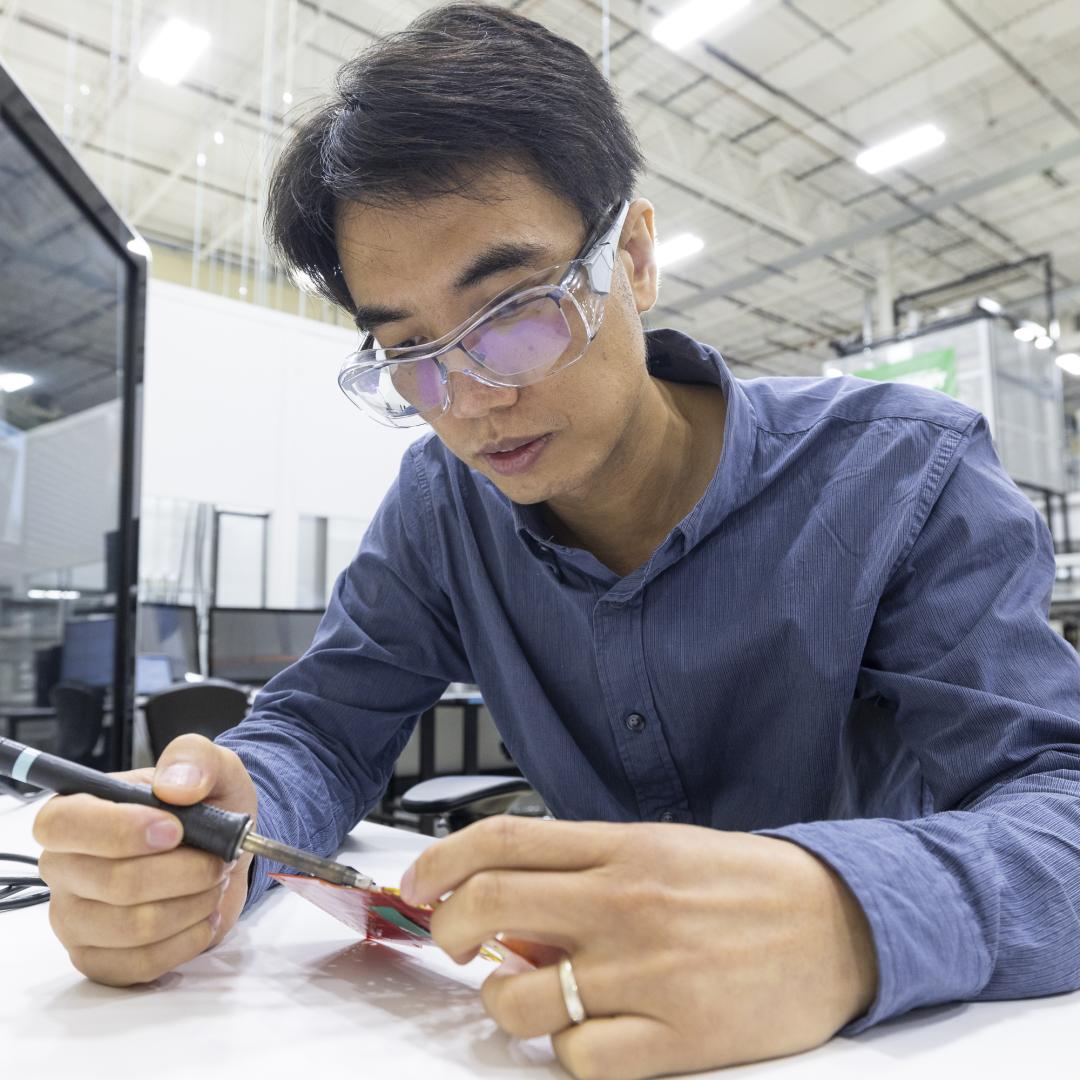
Filter News
Area of Research
- (-) National Security (24)
- (-) Supercomputing (55)
- Advanced Manufacturing (4)
- Biology and Environment (19)
- Clean Energy (28)
- Computational Biology (1)
- Computational Engineering (2)
- Computer Science (7)
- Electricity and Smart Grid (1)
- Functional Materials for Energy (1)
- Fusion and Fission (9)
- Fusion Energy (7)
- Materials (65)
- Materials for Computing (5)
- Mathematics (1)
- Neutron Science (102)
- Nuclear Science and Technology (17)
- Nuclear Systems Modeling, Simulation and Validation (1)
- Quantum information Science (1)
News Topics
- (-) Advanced Reactors (2)
- (-) Artificial Intelligence (45)
- (-) Machine Learning (23)
- (-) Mathematics (1)
- (-) Neutron Science (15)
- (-) Physics (8)
- 3-D Printing/Advanced Manufacturing (7)
- Big Data (22)
- Bioenergy (11)
- Biology (14)
- Biomedical (17)
- Biotechnology (3)
- Buildings (4)
- Chemical Sciences (5)
- Climate Change (20)
- Computer Science (104)
- Coronavirus (16)
- Critical Materials (3)
- Cybersecurity (23)
- Decarbonization (6)
- Energy Storage (9)
- Environment (25)
- Exascale Computing (22)
- Frontier (28)
- Fusion (2)
- Grid (11)
- High-Performance Computing (40)
- Isotopes (1)
- Materials (16)
- Materials Science (17)
- Microscopy (7)
- Molten Salt (1)
- Nanotechnology (11)
- National Security (35)
- Net Zero (1)
- Nuclear Energy (8)
- Partnerships (5)
- Polymers (2)
- Quantum Computing (19)
- Quantum Science (25)
- Security (14)
- Simulation (14)
- Software (1)
- Space Exploration (3)
- Summit (42)
- Sustainable Energy (12)
- Transportation (8)
Media Contacts
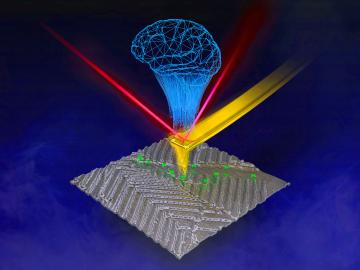
Researchers at ORNL are teaching microscopes to drive discoveries with an intuitive algorithm, developed at the lab’s Center for Nanophase Materials Sciences, that could guide breakthroughs in new materials for energy technologies, sensing and computing.
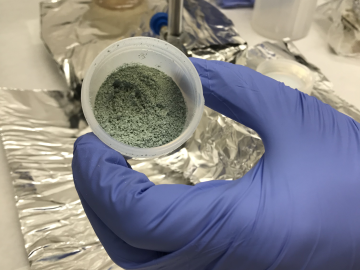
ORNL researchers used the nation’s fastest supercomputer to map the molecular vibrations of an important but little-studied uranium compound produced during the nuclear fuel cycle for results that could lead to a cleaner, safer world.
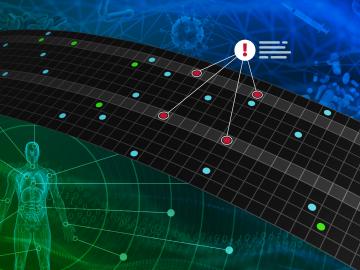
A team of researchers has developed a novel, machine learning–based technique to explore and identify relationships among medical concepts using electronic health record data across multiple healthcare providers.

A study led by researchers at ORNL could help make materials design as customizable as point-and-click.
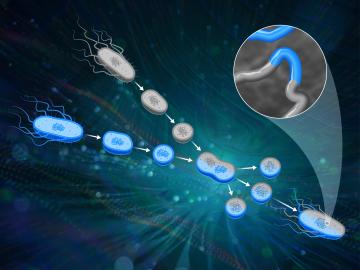
ORNL scientists had a problem mapping the genomes of bacteria to better understand the origins of their physical traits and improve their function for bioenergy production.

A force within the supercomputing community, Jack Dongarra developed software packages that became standard in the industry, allowing high-performance computers to become increasingly more powerful in recent decades.

A study led by researchers at ORNL used the nation’s fastest supercomputer to close in on the answer to a central question of modern physics that could help conduct development of the next generation of energy technologies.
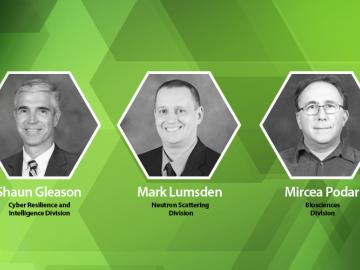
Three ORNL scientists have been elected fellows of the American Association for the Advancement of Science, or AAAS, the world’s largest general scientific society and publisher of the Science family of journals.

A team of scientists led by the Department of Energy’s Oak Ridge National Laboratory and the Georgia Institute of Technology is using supercomputing and revolutionary deep learning tools to predict the structures and roles of thousands of proteins with unknown functions.
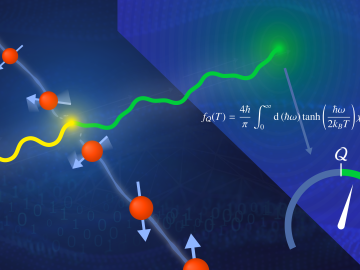
A team led by the U.S. Department of Energy’s Oak Ridge National Laboratory demonstrated the viability of a “quantum entanglement witness” capable of proving the presence of entanglement between magnetic particles, or spins, in a quantum material.