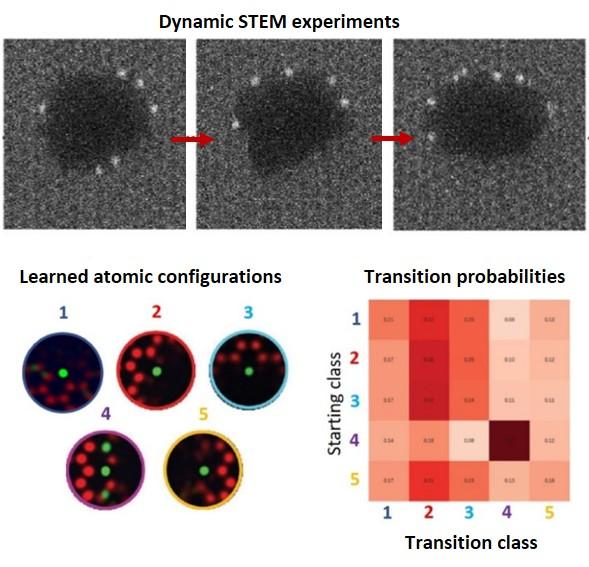
(Top) Dynamic STEM experiments capture Si impurities moving at the edge of a hole in graphene.
(Bottom) Machine learning analysis of dynamic STEM data allows unsupervised identification of structural building blocks and determination of the individual kinetic constants for their transformations. Markov transition matrix for the combined classes of Si–C edge configurations.
(Bottom) Machine learning analysis of dynamic STEM data allows unsupervised identification of structural building blocks and determination of the individual kinetic constants for their transformations. Markov transition matrix for the combined classes of Si–C edge configurations.
Scientific Achievement
Developed a machine learning method to map the chemical transformation networks from scanning transmission electron microscopy movies.
Significance and Impact
Mapping chemical reactions in solids, including identification of relevant structural units and their transformations, enables fundamental understanding of the chemistry and reaction pathways of systems for energy and information technology applications.