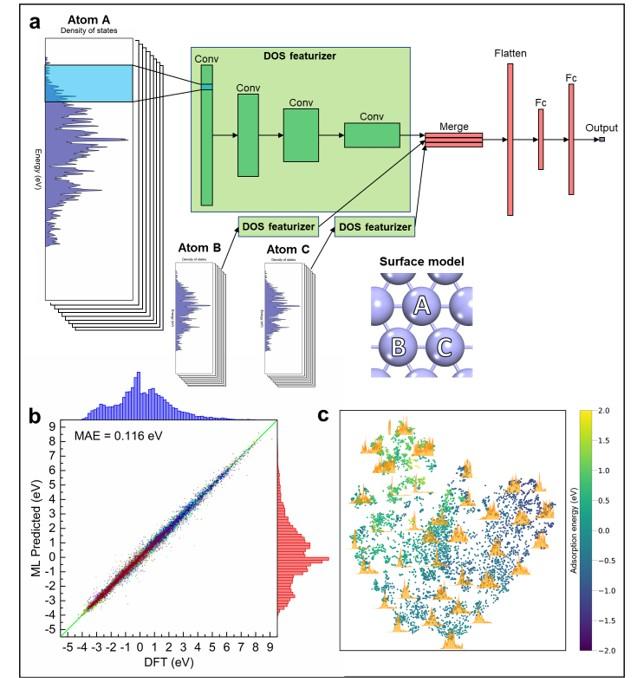
(a) General schematic of the DOSnet machine learning architecture. (b) Prediction parity plot for DOSnet on 37,000 adsorption energies. (c) Visualization of electronic feature space obtained from DOSnet convolutional filters.
Scientific Achievement
A machine learning method, DOSnet, was used to extract key features from the electronic density of states and provide accurate predictions of adsorption energies for catalysis.
Significance and Impact
DOSnet provides an electronic latent space, which can be freely explored without additional ab initio calculations, greatly accelerating materials discovery and design.
Research Details
- A 1D convolutional neural network model was used to featurize the electronic density of states.
- DOSnet can accurately predict adsorption energies with accuracies of ~0.1 eV when tested on a diverse dataset containing 2000 metal alloy surfaces and 11 adsorbate types.
Victor Fung, Guoxiang Hu, P. Ganesh, and Bobby G. Sumpter, “Machine Learned Features from Density of States for Accurate Adsorption Energy Prediction," Nature Communications (2021). DOI: 10.1038/s41467-020-20342-6