
Filter News
Area of Research
News Topics
- (-) Machine Learning (10)
- 3-D Printing/Advanced Manufacturing (12)
- Advanced Reactors (4)
- Artificial Intelligence (14)
- Big Data (9)
- Bioenergy (19)
- Biology (28)
- Biomedical (6)
- Biotechnology (3)
- Buildings (16)
- Chemical Sciences (15)
- Clean Water (5)
- Climate Change (26)
- Composites (3)
- Computer Science (20)
- Coronavirus (9)
- Critical Materials (4)
- Cybersecurity (7)
- Decarbonization (21)
- Element Discovery (1)
- Energy Storage (25)
- Environment (36)
- Exascale Computing (8)
- Fossil Energy (1)
- Frontier (10)
- Fusion (7)
- Grid (13)
- High-Performance Computing (16)
- Hydropower (8)
- Irradiation (1)
- Isotopes (4)
- ITER (2)
- Materials (37)
- Materials Science (16)
- Mercury (1)
- Microscopy (13)
- Nanotechnology (9)
- National Security (17)
- Net Zero (2)
- Neutron Science (12)
- Nuclear Energy (10)
- Partnerships (8)
- Physics (10)
- Polymers (5)
- Quantum Computing (7)
- Quantum Science (9)
- Security (4)
- Simulation (6)
- Space Exploration (4)
- Summit (7)
- Sustainable Energy (25)
- Transformational Challenge Reactor (2)
- Transportation (10)
Media Contacts
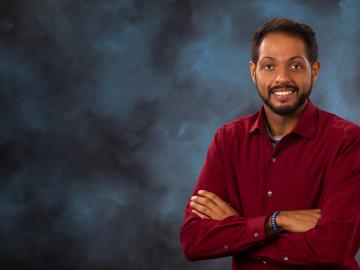
Having lived on three continents spanning the world’s four hemispheres, Philipe Ambrozio Dias understands the difficulties of moving to a new place.
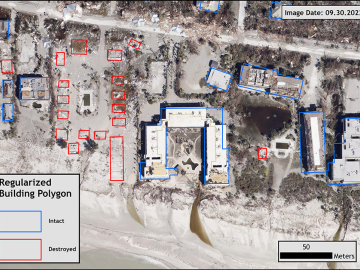
Over the past seven years, researchers in ORNL’s Geospatial Science and Human Security Division have mapped and characterized all structures within the United States and its territories to aid FEMA in its response to disasters. This dataset provides a consistent, nationwide accounting of the buildings where people reside and work.
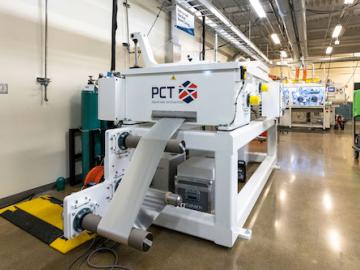
Researchers at the Department of Energy’s Oak Ridge National Laboratory and their technologies have received seven 2022 R&D 100 Awards, plus special recognition for a battery-related green technology product.

The Frontier supercomputer at the Department of Energy’s Oak Ridge National Laboratory earned the top ranking today as the world’s fastest on the 59th TOP500 list, with 1.1 exaflops of performance. The system is the first to achieve an unprecedented level of computing performance known as exascale, a threshold of a quintillion calculations per second.
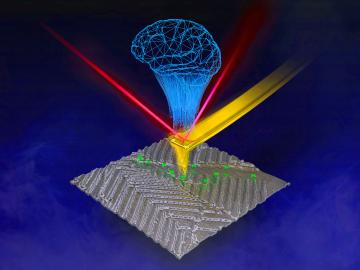
Researchers at ORNL are teaching microscopes to drive discoveries with an intuitive algorithm, developed at the lab’s Center for Nanophase Materials Sciences, that could guide breakthroughs in new materials for energy technologies, sensing and computing.
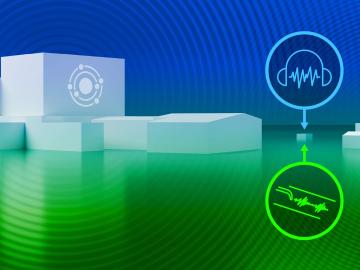
An Oak Ridge National Laboratory team developed a novel technique using sensors to monitor seismic and acoustic activity and machine learning to differentiate operational activities at facilities from “noise” in the recorded data.
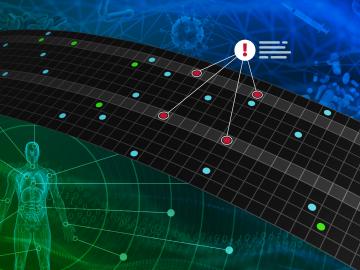
A team of researchers has developed a novel, machine learning–based technique to explore and identify relationships among medical concepts using electronic health record data across multiple healthcare providers.
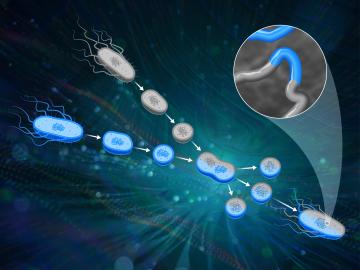
ORNL scientists had a problem mapping the genomes of bacteria to better understand the origins of their physical traits and improve their function for bioenergy production.
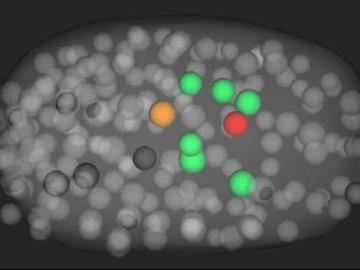
Scientists have developed a novel approach to computationally infer previously undetected behaviors within complex biological environments by analyzing live, time-lapsed images that show the positioning of embryonic cells in C. elegans, or roundworms. Their published methods could be used to reveal hidden biological activity.

A team of scientists led by the Department of Energy’s Oak Ridge National Laboratory and the Georgia Institute of Technology is using supercomputing and revolutionary deep learning tools to predict the structures and roles of thousands of proteins with unknown functions.