Topic:
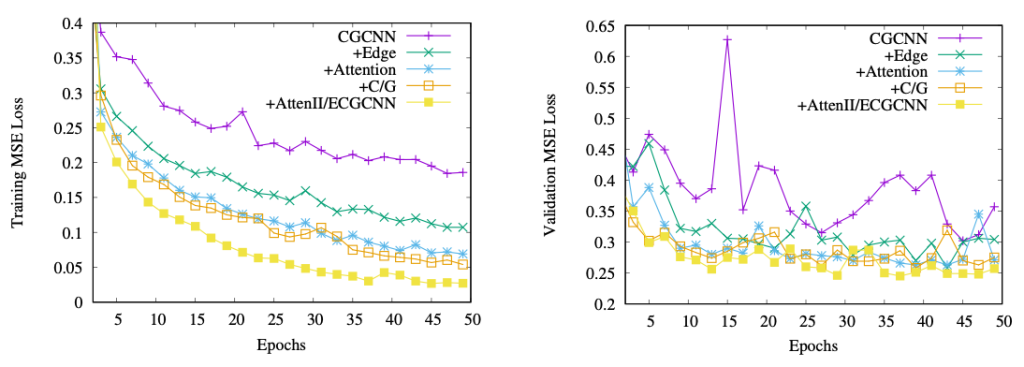
Training and validation losses for optimizations introduced in ECGCNN in comparison to CGCNN
We have constructed an enhanced crystal graph convolution neural network (ECGCNN) to predict the carbon dioxide ad- sorption properties of MOF crystalline nanoporous materials. Our ECGCNN framework matches the best reported performance of classical machine learning methods, which re- quire the costly computation of more than a hundred chemical and geometric material features. Future work could include greater gas molecule specificity within the network, as well as the training of the model to chemical process figures- of-merit for increased efficiency.
Citation and DOI:
Prediction of CO2 Adsorption in Nano-Pores with Graph Neural Networks, Workshop on Deep Learning on Graphs: Methods and Applications, AAAI22
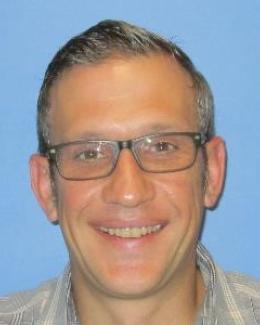